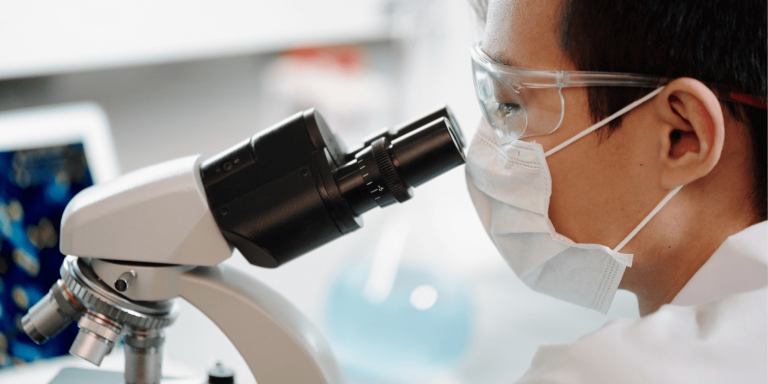
The use of machine learning methods for prediction and forecasting has become widespread in the quantitative sciences. However, there are many known methodological pitfalls, including data leakage; for Professor Arvind Narayanan and his PhD student Sayash Kapoor, a reproducibility crisis is brewing. They held a workshop on July 28 to highlight the magnitude of this crisis, identify the root causes of observed reproducibility, and find solutions to this problem.
Prior to this workshop, Arvind Narayanan and Sayash Kapoor had been systematically examining reproducibility problems in ML-based science, their study “Leakage and Reproducibility Crisis in ML-based Science” was actually published on arXiv in February 2020.
To investigate the impact of reproducibility errors and the effectiveness of model fact sheets, their work focused on reproducibility in an area where complex ML models are thought to vastly outperform older statistical models such as logistic regression (LR): predicting civil war. They were able to find that papers claiming superior performance of complex ML models over LR models do not replicate due to data leakage and that complex ML models do not really perform any better than decades-old LR models.
They found that other researchers had identified 329 papers about 17 scientific fields in which poorly implemented machine learning produced questionable results. In the field of political science, one claimed that it was possible to predict when a civil war will break out with more than 90% accuracy, using AI.
The Reproducibility Crisis Workshop
The two Princeton researchers decided to hold an online workshop. Hosted by the Center for Statistics and Machine Learning at Princeton University, the online workshop aimed to highlight the size and scope of the crisis, identify the root causes of the observed reproducibility failures, and move toward solutions.
They expected about 30 participants, but more than 1,500 people signed up, a surprise they say suggests that machine learning problems in science are widespread.
During the event, guest speakers cited several examples of where AI is being misused in fields such as medical and social sciences:
Michael Roberts, a senior research associate at the University of Cambridge, discussed the problems with dozens of papers claiming to use machine learning to combat COVID-19, particularly when the data was skewed because it had been exposed to different imaging machines.
Jessica Hulman, an associate professor at Northwestern University, compared the problems with studies using machine learning to prove the unreplicable phenomenon of major findings in psychology. She said, ” In both cases, researchers risk using too little data and misinterpreting the statistical significance of the results.”
Momin Malik, a data scientist at the Mayo Clinic, talked about his work tracking problematic uses of machine learning in science. He said, ” In addition to common mistakes in implementing the technology, researchers sometimes apply machine learning when it’s not the right tool for the job.” In particular, he cited an example of machine learning that produced misleading results – Google Flu Trends, a tool developed in 2008 to more quickly identify flu outbreaks from logs of search queries typed by Internet users.
To him, Google got positive publicity for the project but failed spectacularly to predict the course of the 2013 flu season. An independent study later concluded that the model was based on seasonal conditions that had nothing to do with the spread of the flu. “You can’t put everything into one big machine learning model and see what comes out,” Momin Malik explained.
For some of the workshop participants, not all scientists can master machine learning, especially given the complexity of some of the problems.
For example, Amy Winekoff, a data scientist at the Princeton Center for Information Technology Policy, says that while it’s important for scientists to learn good software engineering principles, master statistical techniques and take the time to maintain data sets, it shouldn’t come at the expense of the field.
She stated:
“For example, we don’t want schizophrenia researchers to know too much about software engineering, but too little about the causes of the disorder.”
She suggests that greater collaboration between scientists and computer scientists could help strike the right balance.
Momin Malik, meanwhile, assures:
“The overall lesson is that it’s not right to do everything with machine learning. Despite the rhetoric, the hype, the successes and hopes, it remains a limited approach.”
For Sayash Kapoor, it’s important that the scientific community start thinking about the issue.
He states:
“Machine learning-based science is still in its infancy. But it is urgent – it can have very harmful consequences in the long run.”
Translated from Quelles sont les causes de la crise de reproductibilité dans le domaine de la science basée sur le ML ?