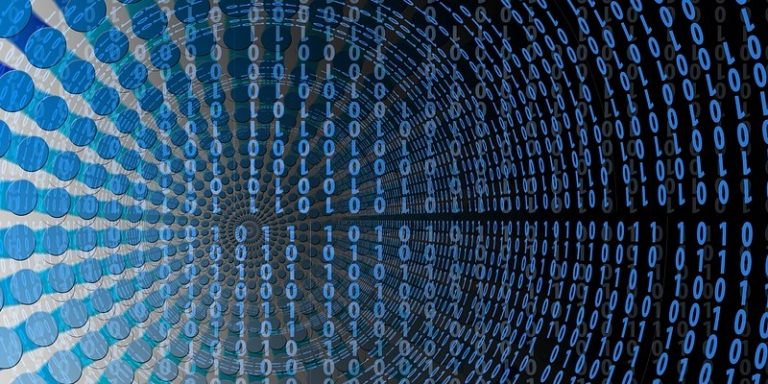
In the framework of research on artificial intelligence, a team of three researchers from the Massachusetts Institute of Technology (MIT) published a paper at the end of March, showing that the databases used by the algorithms to analyze a query were not really reliable.
It was while testing object and animal recognition algorithms that Curtis Northcutt, Jonas Mueller and Anish Athalye, all three researchers at MIT, noticed that these databases contained many errors. These algorithms, if they work properly, should recognize the object or animal from any image sent to them and tell the user what it is. But during the tests, some results seemed to be out of place: a picture of a crab was described as a lobster, a frog was recognized by the algorithm as a cat and a can opener, after analysis, became a nutcracker.
The researchers therefore decided to examine a dozen databases by designing an algorithm based on their own databases and to compare it with the tested databases. The result was clear, the error rates can vary from 0.54% error for the CIFAR-10 database to more than 10% for Quick!Draw! with an average error rate of 3.4%. What is certain is that the absolute zero in error does not exist. However, continuing to mislabel visual, audio, video or textual data could potentially slow down research in the field of automatic recognition.
Translated from Trois chercheurs du MIT ont découvert que des bases de données comportaient certaines erreurs d’appréciation