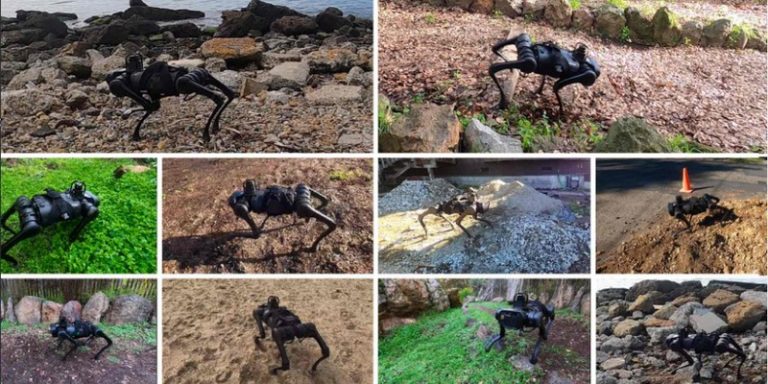
Through Rapid Motor Adaptation (RMA ),Facebookis making progress in adapting robots to their environment in order to develop their motor skills.
Adapting robots to an environment just like a human can do in everyday life
Humans can walk with relative ease over rocks, through mud, up and down hills, or jump on trampolines or balance on a rather soft surface. This is made possible by our powerful yet flexible muscles that adapt to the conditions of the environment in which we find ourselves.
A research team consisting of experts from FAIR, the University of Berkley and Carnegie Mellon University used this observation in humans to design an AI model called Rapid Motor Adaptation (RMA) that allows legged robots to adapt in real time to a variety of environments.
Until now, legged robots were either completely hand-coded for the environments they encountered or experts made them learn to navigate their environments through a combination of hand-coding and machine learning techniques.
An adaptation model designed using reinforcement learning and supervised learning
RMA uses a combination of two AI-related methods in order to function:
- First module – Reinforcement learning: the tool exploits reinforcement learning by using information it selects from the different environments it may encounter (friction, weight and shape of the payload, etc.). The robot adapts to the ground’s adherence as well as to its inclination.
- Second module – Adaptation module: it was not possible, according to the researchers, to rely solely on reinforcement learning, as it is not possible to know in advance what real elements the robot might encounter on its way. Supervised learning was used by the research team as an “adaptation module” so that the robot could adapt to these hazards. This module was trained using supervised learning.
The two modules work hand in hand and asynchronously: the first module runs quickly while the second takes longer to set up.
An efficient robot that adapts to environments thanks to AI, even when carrying a payload
A battery of tests has been carried out by the research team coordinating the project. They show that an RMA-enabled robot outperforms so-called “alternative” systems (those that are coded specifically for an environment) when walking on different surfaces or slopes, encountering obstacles, or when given different payloads to carry.
The robot used in the experiments and equipped with the RMA was able to walk on sand, mud, hiking trails, tall grass and a pile of dirt without a single failure. In 70% of the tests performed, the robot successfully walked down steps along a hiking trail, and in 80% of the tests performed, it successfully navigated a pile of cement and piles of rocks. A high success rate was maintained even when moving with a payload of twelve kilograms, a mass as heavy as its own weight.
Beyond the robotic possibilities, the researchers say their work could be useful for the design of AI models capable of adapting to many situations and challenges, complex in real time, and which would take into account, like the robots, all the characteristics of the environment to fully appropriate the environment.
Translated from L’apprentissage supervisé et par renforcement pour aider les robots à s’adapter à tout type d’environnement