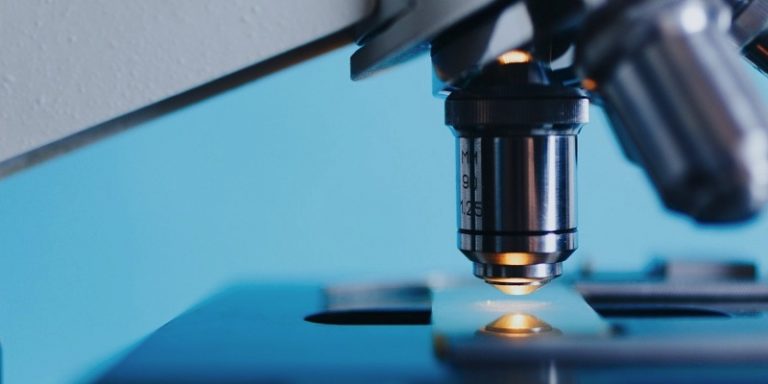
A rare hereditary disease, Huntington’s disease affects about 6,000 people in France. It results in neurological degeneration leading to significant motor, cognitive and psychiatric disorders, loss of autonomy and death. Numerous teams are working on diagnosis and testing methods for Huntington’s disease as well as on therapeutic approaches.
Shape deformation analysis reveals the temporal dynamics of cell-type-specific homeostatic and pathogenic responses to mutant huntingtin – Lucile Megret, Barbara Gris, Satish Sasidharan Nair, Jasmin Cevost, Mary Wertz, Jeff Aaronson, Jim Rosinski, Thomas F Vogt, Hilary Wilkinson, Myriam Heiman, Christian Neri, eLife, 23 February 2021. DOI: https: //doi.org/10.7554/eLife.64984
The research team (Sorbonne University/Inserm/CNRS), led by Christian Néri, Inserm research director at the Institute of Biology Paris-Seine, in collaboration with MIT (USA), has recently shown that the loss of compensation mechanisms and neuronal resilience to Huntington’s disease would be the main driver of the evolution of this disease at the molecular level. Published in eLife on 23 February 2021 and based on an original machine learning method for the precise analysis of large volumes of genomic data obtained in models of the disease, this work suggests that restoring neuronal resilience is an important therapeutic avenue for early intervention to combat this disease.
The cells in our body are naturally able to resist disease through cellular homeostasis, a series of adaptive mechanisms that repair cellular damage, involving hundreds of genes that make our cells resilient. Although homeostasis holds great potential for protecting neurons in aging and neurodegenerative diseases, the importance and dynamics of homeostatic mechanisms in neurodegenerative diseases have remained elusive due to the difficulties of studying different cell types in the mammalian brain.
Recently, genomic screening technologies have been used to investigate how different brain cells use hundreds of genes to modulate neurodegenerative processes. The complexity of this data, however, makes it difficult to analyze. In collaboration with Myriam Heiman’s team at MIT who obtained these data in the USA and the Laboratoire Jacques-Louis Lions (Sorbonne University/CNRS), the research team has developed a mathematical approach capable of precisely identifying the groups of genes used in the brains of mouse models to counter the toxic effects of mutant huntingtin, the gene for Huntington’s disease (HD), over time and in several types of neurons that make up the striatum, an area of the brain that is heavily affected by Huntington’s disease.
Scientists have tested whether neuronal death in this disease is primarily due to the enhancement of pathogenic responses or the loss of homeostatic responses. Answering this question could significantly change our views on how best to counter the progression of Huntington’s disease. It could provide biomarkers as to whether a therapeutic intervention protects the brain by blocking pathogenesis or increasing homeostasis, thereby improving the accuracy of preclinical studies.
To do this, they developed Geomic, a machine learning method based on the geometry of genomic data that allows them to analyze the shape (curves, surfaces) of gene expression and to map the temporal dynamics of homeostatic and pathogenic responses. Unexpectedly, this map shows that most pathogenic responses are attenuated over time and, moreover, that most homeostatic responses decrease.
These results suggest that neuronal death in Huntington’s disease is mainly due to the loss of homeostatic molecular responses and not to the enhancement of pathogenic molecular responses, underlining the importance of homeostatic processes in the evolution of the disease.
These results provide a conceptual framework to explore the development of therapeutic strategies focused on restoring the resistance capacity of brain cells to Huntington’s disease. They provide a roadmap for selecting therapeutic targets to restore neuronal resilience and biomarkers to monitor whether emerging drugs can engage homeostatic mechanisms to be effective, and to use these tools in experimental models of this disease. The findings pave the way for applications of Geomic to ‘omics’ data analysis in several other diseases, including other neurodegenerative diseases.