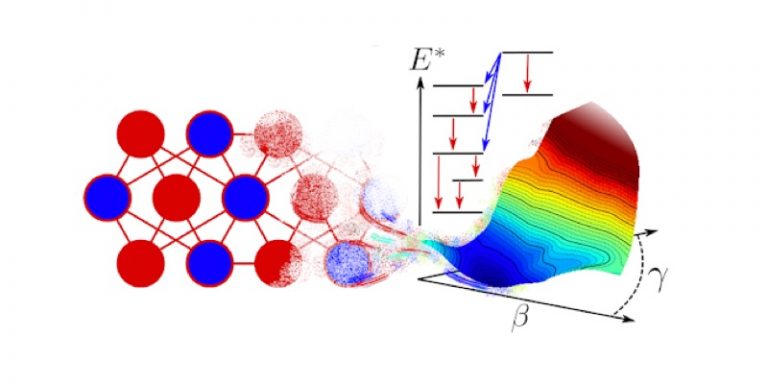
The work of Raphaël-David Lasseri, David Regnier, Jean-Paul Ebran and Antonin Penon, theoreticians at the CEA, Irfu at the DRF (Espace de Structure Nucléaire Théorique) and the Nuclear Physics Department at the DAM, was published this year in the journal Physical Review Letters , the article is among the suggestions of the editor. The researchers have developed an artificial intelligence for predicting the properties of the atomic nucleus. They have simulated the properties of more than 1800 atomic nuclei using an algorithm trained on a subset of only 210 nuclei.
These 210 kernels were chosen automatically by the AI using a so-called active learning approach, a first. This is a major advance compared to previous approaches that were limited to the prediction of a single observable (measurable physical quantity, such as mass) and had a very low predictive range. The results obtained are of a precision comparable to that of calculations based on the state of the art techniques used in theoretical nuclear physics, and this in a significantly reduced calculation time (a gain ranging from a factor of 10 to a factor of 10³ depending on the type of result desired).
Understanding the structure and limit of existence of atomic nuclei is an essential and difficult issue. It is however necessary to determine the origin of heavy elements in our galaxy as well as to discover new super-heavy elements. Motivated by the rapid progression of machine learning over the last 10 years, the study aimed to examine, for the first time, the ability of an AI to grasp the physics of low-energy nuclear structures as a whole.
Energy Density Functionals: A Proven Theoretical Approach
Among the various theoretical approaches to provide a universal description of nuclear observables, such as the mass, radius or excitation energy spectrum of a nucleus, only the Energy Density Functional (EDF) framework is currently able to provide a complete and accurate description of the properties of the atomic nucleus.
This theoretical approach describes the interactions between nucleons, i.e. the protons and neutrons making up the nucleus, as a function of the density of the system. In particular, the most recent approaches, known as Multi-Referenced EDF (MR-EDF), provide accurate and universal observable predictions at the expense of a very consequent increase in computation time.
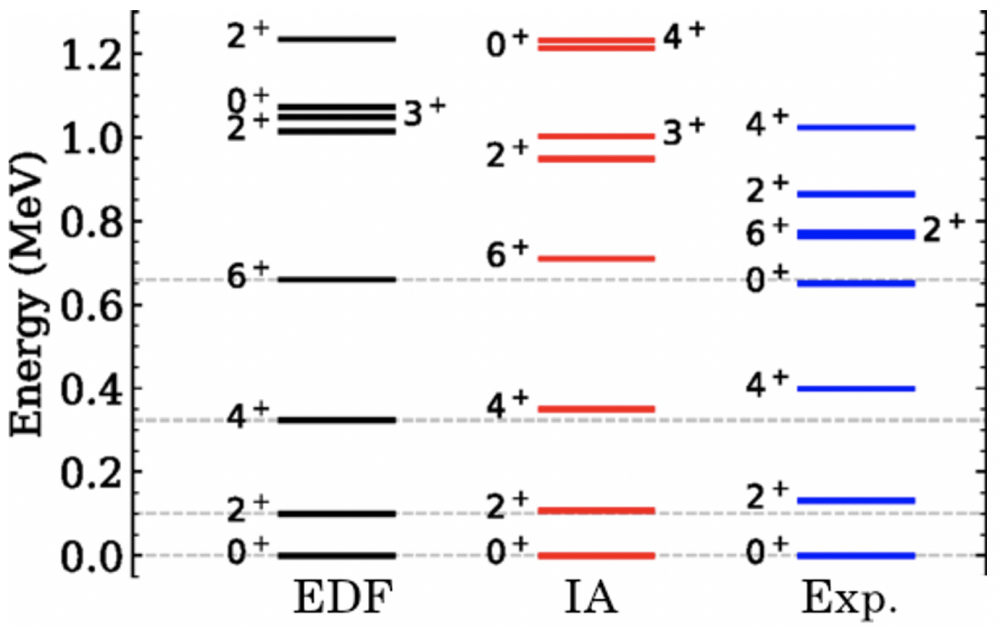
Results
For the first time ever, an algorithm consisting of a neural network committee was able to estimate the low-energy structure of all nuclei. Remarkable performances are obtained, e.g. a value of the mean energy deviation of the EDF fundamental states of only 716 keV (rms) (i.e. less than 0.5 % error for Osmium 178).
This for a training set of only 210 nuclei automatically selected as the most representative of the physics of the whole nuclear map. Moreover, the distribution of the automatically selected nuclei is globally consistent with the physical intuition with an important representation of light nuclei and nuclei close to drip-lines.
Finally, it is important to note that once the network has been trained on all the drive cores, the prediction of all the observables on the whole map takes only a few milliseconds compared to several hundred CPU hours in case of direct EDF calculation. It is therefore now possible to perform only ~200 EDF calculations before being able to generalize the results of a functional on the whole map while keeping a very good accuracy compared to the experiment.
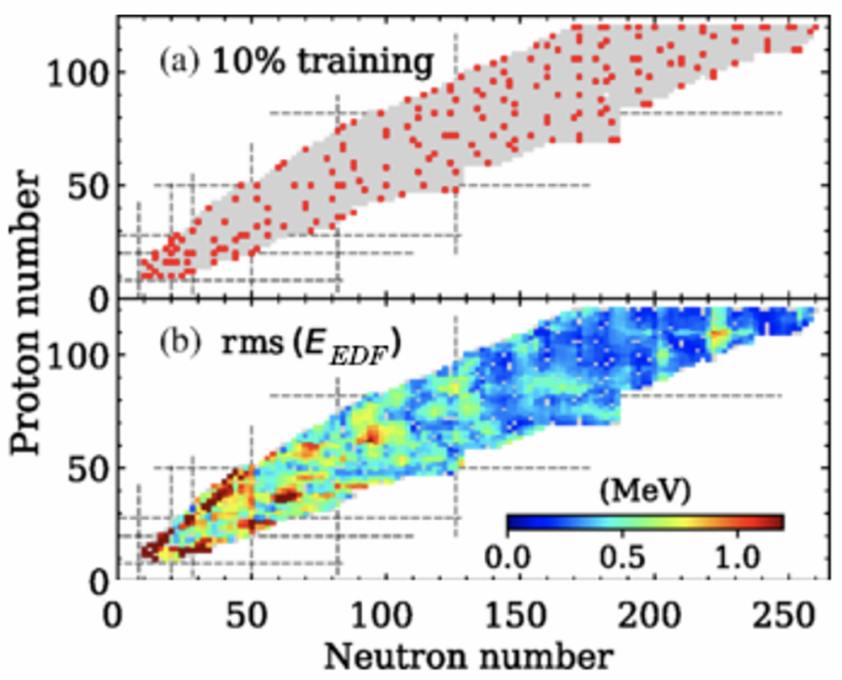
A promising future for IA approaches to nuclear structure
This work now paves the way for many improvements and uses of AI algorithms for the theoretical description of atomic nuclei. Firstly, in view of the consequent gain in computing time, it is now possible to use these algorithms to rapidly build new EDFs taking into account a large number of experimental observables (masses, radii, but also characteristics of the ground state and excited states).
This approach is particularly important for astrophysical applications and is already being evaluated by a Franco-Belgian collaboration. Beyond these direct applications, it is also becoming possible to use neural networks to provide good quality starting points for nuclear dynamics calculations, particularly for the study of fission.
Finally, the success of this approach is a first proof of principle that a neural network committee is capable of coding several correlated aspects of nuclear deformation. The neural networks involved probably have a satisfactory non-trivial internal representation of the physics of the system. The study of this representation may reveal new physical concepts that are captured during learning.
On a larger scale, the information that can be extracted from an AI algorithm about the representation of a complex physical problem is a key issue at the intersection of physics and representation theory.
Reference
1] R&D. Lasseri, D. Regnier, J-P. Ebran, and A. Penon, Phys. Rev. Lett. 124, 162502 (2020)
Contacts
Raphaël Lasseri
David Regnier
Translated from Des chercheurs ont développé une intelligence artificielle permettant la prédiction des propriétés du noyau atomique