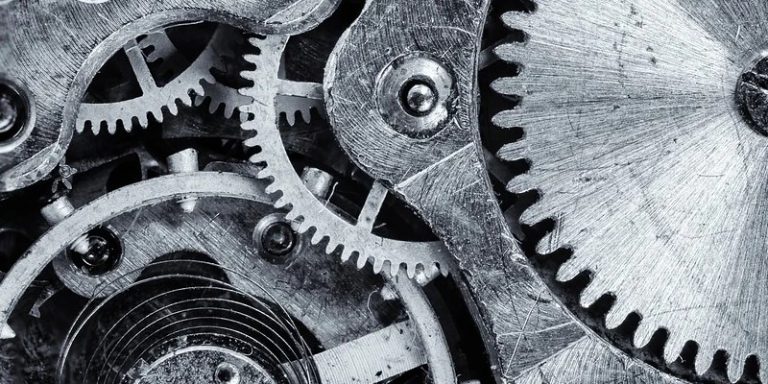
Many researchers use machine learning to train computers to recognize objects visually, but few allow themselves to use this learning method for mechanical parts: brakes, clutches, engines, nuts, bolts, gearboxes, etc. And for good reason, the available data sets are scarce. To remedy this, a team of mechanical engineers from Purdue University has developed an open source database of over 58,000 3D mechanical parts. The goal: to help researchers use this database to train their machine learning models designed to work with real mechanical parts.
Engineering team’s initiative to promote the adoption of machine learning in mechanics
Purdue University mechanical engineering professor Karthik Ramani’s team announced that they have designed a database that allows researchers to train their machine learning or deep learning models for applications related to mechanical parts such as bearings. Speaking about his team’s desire to design a dataset related to these mechanical tools, he said:
“We are in the era of deep learning where we use computers to search for things visually. But no one is focusing on the parts that make up the machines: pipes, bearings, motors, washers, nuts and bolts, etc. These are the things that are important to us as engineers and manufacturers. We want to be able to point a camera at a real-world part and have the computer tell us everything about that part or its design.”
Back in 2004, Professor Ramani’s engineering team had already done some experiments around visual search of mechanical parts. However, at that time, machine learning and the computing power that processors offered were not sufficiently advanced. The next fifteen years allowed researchers in the artificial intelligence community to learn more about AI models and database design: creating a robust dataset depends on quantity, but also on quality.
For Karthik Ramani, there are many challenges in the world of machine learning and deep learning:
“There are many challenges in machine learning. But you can’t meet those challenges unless you know how to test the performance of what you’ve done. We’re proud to have created the first large-scale reference dataset of annotated 3D mechanical components, and we hope to advance visual search in the deep learning era.”
A comprehensive database of 3D mechanical parts
To design the database, the engineering team partnered with TraceParts, a French company specializing in 3D models and 2D drawings, and collaborated with assistant professor Qixing Huang from the University of Texas at Austin to scour other databases for 3D models similar to what the engineers want to design. Karthik Ramani adds:
“Deep learning is data-intensive: it takes a lot of examples for the computer to learn everything they need to perform tasks and know how things relate to each other. That meant we needed a lot of 3D models of parts that also required underlying technical classification.”
Together, they managed to design a database of 58,696 mechanical parts and components. Each 3D part was labeled according to a 68-class taxonomy based on the International Organization for Standardization’s (ISO) International Classification of Standards. Their dataset is open source and has been released for anyone to use. Karthik Ramani spoke about the possibilities of using this database:
“We see many real-world situations that can leverage this technology. Imagine you’re working in maintenance in a factory and you’re replacing part of a machine. You can point a camera at the part, the computer will recognize it and instantly give you all the specs of that part – what it’s called, what it connects to and where it’s physically stored in the factory. This could even be possible with augmented reality glasses: you can instantly have the entire visual catalog of your company at your fingertips and learn how to fix things or order parts.”
Translated from L’Université Purdue conçoit une base de données de pièces mécaniques 3D pour les modèles ML/DL