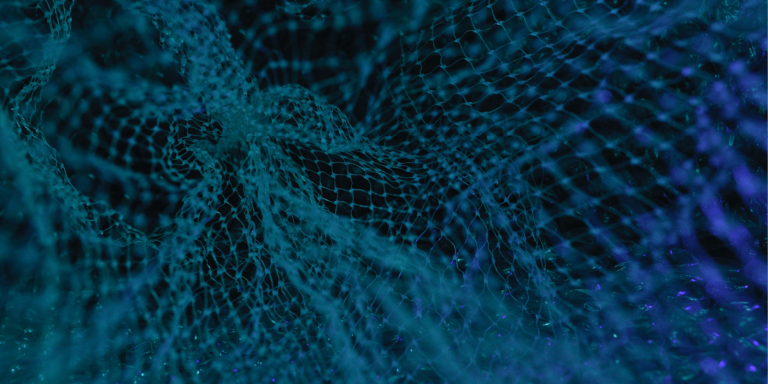
Proteins are present in all living cells where they perform essential functions. Understanding the relationship between a protein’s amino acid sequence, for example, its domains and its structure or function is the subject of much scientific research. A team of researchers from Google, BigHat Biosciences, the University of Cambridge, the European Molecular Biology Laboratory, the Francis Crick Institute and MIT used deep learning to predict protein function. Their study, “Using Deep Learning to Annotate the Protein Universe,” was published in Nature Biotechnology.
Computational prediction of protein structure from amino acid sequences has made great strides, with DeepMind’s AlphaFold model or ProfileView ‘s computational classification approach being examples.
Existing approaches have successfully predicted the function of hundreds of millions of proteins, however, the functions of many others are still unknown, a study published in Nature pointed out that 1/3 of microbial proteins are not reliably annotated. As the volume and diversity of protein sequences in public databases grows, accurately predicting the function of highly divergent sequences is a paramount challenge.
Using deep learning
to annotate the protein universe
To infer protein function directly from sequences, the database of 137 million proteins and nearly 18,000 protein family classifications, Pfam, which contains many highly detailed computational annotations describing the function of a protein domain, such as the globin and trypsin families, is very often used.
The team trained deep learning models to accurately predict functional annotations for unaligned amino acid sequences from 17,929 families in the Pfam database, which incidentally added about 6.8 million entries to the Pfam set, roughly the sum of the progress made over the past decade.
Their approach is based on dilated convolutional neural networks (CNNs), which are suitable for modeling non-local pairwise interactions of amino acids and can be run on modern ML hardware such as GPUs. Thus, they trained one-dimensional CNNs to predict protein sequence classification, which they named ProtCNN, as well as a set of independently trained ProtCNN models, called ProtENN.
Results of the study
ProtENN achieved 99.8% accuracy, superior to both comparative representations (99.2%) and the BLAST method (98.3%). For the classification of low-resource family members, the representation-comparison method achieved 85.1% accuracy.
Combining deep models with existing methods significantly improved remote homology detection, suggesting that deep models learn complementary information. For the team, these results suggest that deep learning models will be a central component of future protein annotation tools.
To encourage further research in this direction, they have published the ProtENN model and an interactive paper that allows the user to enter a sequence and get results for a predicted protein function in real time, in the browser, with no configuration required.
Article sources:
“Using deep learning to annotate the protein universe,” nature biotechnology,
doi.org/10.1038/s41587-021-01179
Authors:
Maxwell L. Bileschi,Google Research, Cambridge, MA, USA;
David Belanger, Google Research, Cambridge, MA, USA;
Drew H. Bryant, Google Research, Cambridge, MA, USA;
Theo Sanderson, Google Research, Cambridge, MA, USA; Francis Crick Institute, London, UK;
Brandon Carter, MIT Computer Science and Artificial Intelligence LABORATORY, Cambridge, MA, USA;
D. Sculley, Google Research, Cambridge, MA, USA;
Alex Bateman, European Molecular Biology Laboratory, European Bioinformatics Institute (EMBL-EBI), Hinxton, UK;
Mark A. DePristo, Google Research, Cambridge, MA, USA; BigHat Biosciences, San Mateo, CA, USA;
Lucy J. Colwell, Google Research, Cambridge, MA, USA, Department of Chemistry, University of Cambridge, Cambridge, UK
Translated from Prédire la fonction des protéines grâce au deep learning