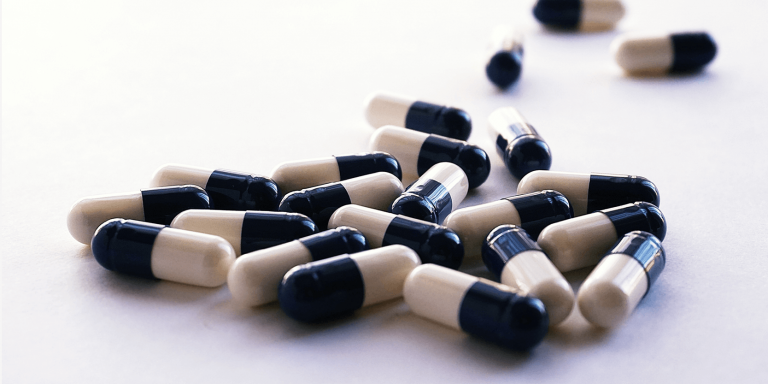
Predicting the structure of proteins is one of the challenges of biological research. The MIT Technology Review relayed an announcement from Deepmind about its AI tool, the AlphaFold program. In it, the company said it had successfully predicted the structure of almost every protein known to scientists and is offering free access to its database of more than 200 million proteins to anyone. At the same time, MIT researchers published the study “Benchmarking AlphaFold-enabled molecular docking predictions for antibiotic discovery” which points out that improvements will be needed to take full advantage of the protein structures provided by AlphaFold.
Deepmind proposed a 1st version of AlphaFold, an AI system for protein structure prediction at the CASP (Critical Assessment of protein Structure Prediction) in 2018, it obtained the 1st place as AlphaFold 2, the second version to which we also dedicated an article in our paper magazine ActuIA N°3, did in 2020.
According to Deepmind, AlphaFold, its AI system that predicts the 3D structure of a protein from its amino acid sequence, regularly achieves an accuracy that is competitive with experience.
In 2021, she published the scientific paper and source code explaining how she created this AI system and partnered with the EMBL European Bioinformatics Institute (EMBL-EBI) to create the AlphaFold DB platform to make these predictions freely available to the scientific community. The latest version of the database contains over 200 million entries, providing broad coverage of UniProt, the standard repository of protein sequences and annotations.
AlphaFold DB is currently focused on the use case validated in CASP14: predicting the structure of a single protein chain with a natural sequence. Deepmind and EMBL will continue to update this database.
However, Deepmind concedes that AlphaFold’s system has some limitations. Researchers at MIT sought to analyze these as well as its potential.
The study: comparative analysis of AlphaFold-enabled molecular docking predictions for antibiotic discovery
The team of researchers, led by James Collins, Termeer Professor of Medical Engineering and Science at MIT’s Institute for Medical Engineering and Science (IMES) and Department of Biological Engineering, investigated whether AlphaFold could accurately predict interactions between bacterial proteins and antibacterial compounds, which could lead to the development of new antibiotics.
Efficiently identifying drug mechanisms of action remains a challenge. Computational docking approaches have been widely used to predict drug binding targets. Yet such approaches are dependent on existing protein structures, and accurate structural predictions are only recently available from AlphaFold2.
For this study, the researchers combined AlphaFold2 with molecular docking simulations to predict protein-ligand interactions between 296 proteins spanning the essential proteome of Escherichia coli, and 218 active and 100 inactive antibacterial compounds, respectively, indicating widespread promiscuity of compounds and proteins.
They then compared model performance by measuring the enzymatic activity of 12 essential proteins treated with each antibacterial compound.
This allowed them to confirm widespread promiscuity, but also found that the average area under the receiver operating characteristic curve (atROC) was 0.48, indicating poor model performance. The researchers showed that rescoring docking poses using machine learning -based approaches improves model performance, resulting in auROCs with an average of 0.63. They also found that correction function sets improve prediction accuracy and the ratio of true positive to false positive rates.
Results of the study
The study demonstrated the potential of AI to streamline the selection process of future antibiotics. However, the results suggest that while AlphaFold2 can provide rich structural information, methods to more accurately model protein-ligand interactions are needed to better exploit AlphaFold2 for drug discovery.
Article references:
“Benchmarking AlphaFold-enabled molecular docking predictions for antibiotic discovery” doi.org/10.15252/msb.202211081
Published in the journal ” Molecular Systems Biology” on September 6, 2022.
Authors:
Wong F, Krishnan A, Zheng EJ, Stark H, Manson AL, Earl AM, Jaakkola T, Collins JJ. 18: e11081.
Affiliations:
Felix Wong1,2,3,AartiKrishnan1,2,3, Erica J Zheng3,4, Hannes Stärk5, Abigail L Manson3, Ashlee M Earl3, Tommi Jaakkola5 andJames J. Collins 1,2,3,6
- 1Institutefor Medical Engineering & Science, Massachusetts Institute of Technology, Cambridge, MA, USA
- 2Departmentof Biological Engineering, Massachusetts Institute of Technology, Cambridge, MA, USA
- 3InfectiousDisease and Microbiome Program, Broad Institute of MIT and Harvard, Cambridge, MA, USA
- 4Programin Chemical Biology, Harvard University, Cambridge, MA, USA
- 5ComputerScience and Artificial Intelligence Laboratory, Massachusetts Institute of Technology, Cambridge, MA, USA
- 6WyssInstitute for Biologically Inspired Engineering, Harvard University, Boston, MA, USA.
Translated from Une nouvelle étude du MIT révèle le potentiel et les limites d’AlphaFold 2, la solution d’IA de Deepmind