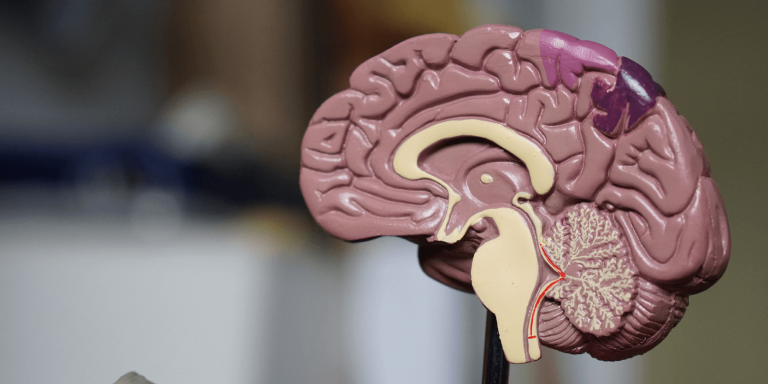
Scientific research is giving us a better understanding of the functioning of the complex organ that is our brain and its 86 to 100 billion neurons. Researchers at Carnegie Mellon University, Albert Einstein College of Medicine and the Champalimaud Foundation have been working for more than 10 years to better understand the flow of communication in the brain and have recently developed a new statistical method, Delayed Latents Across Groups (DLAG). They present their work in the study “Disentangling the flow of signals between populations of neurons” published in Nature Computational Science on August 18.
The brain is essential to the functioning of the human body, and neuronal communication flows allow us to interact with the world around us: taste, touch, sight, hearing and smell are thus transmitted by neurons via sensory flows. Interactions between large collections of neurons that fire simultaneously in the brain allow us to see, hear, smell or walk.
Untangling the simultaneous and bidirectional flow of signals between populations of neurons
To disentangle the signals relayed between brain areas, even when communication between brain areas is bidirectional, the research team developed a new statistical method, called Delayed Latents Across Groups (DLAG).
Evren Gokcen, a graduate student in electrical and computer engineering at Carnegie Mellon, explains:
“The method we developed, DLAG, falls into the broader category of machine learning or statistical methods that examine high-dimensional neural signals. The novel aspect is to identify patterns of activity that are shared between different brain areas.”
He adds:
“For decades, studies have focused on recording one or a handful of neurons from one brain area at a time. But with advances in neural recording technology, the bottleneck has shifted to the ability to analyze and interpret recordings of large populations of neurons from multiple brain areas.”
Activity patterns provide insight into how neurons coordinate their activity with each other. Identifying the patterns of activity involved in communication between different brain areas presents several challenges, one being that this communication usually occurs bidirectionally and simultaneously.
Evren Gokcen explains the team’s approach:
“To make progress in untangled communication, we took advantage of a simple insight: you can’t send signals instantly; it takes some time for information to flow. Video conferencing is a great reference point when thinking about a delay in communication; it’s similar in the brain. With DLAG, we exploit this delay, so if the signal appears first in area A and then in area B, we take that to mean that area A has sent the signal to area B. Using the DLAG method, we can separate the simultaneously relayed signals.”
Study results
The researchers demonstrated that DLAG works well on synthetic datasets similar in scale to current neurophysiological recordings. Next, they simultaneously studied populations recorded in visual areas of primates V1 and V2, where DLAG reveals signatures of bidirectional but selective communication. Their framework lays the groundwork for dissecting the complex flow of signals through populations of neurons, and how this signaling contributes to cortical computation.
DLAG could be used for other neuroscience applications, such as understanding the interaction between different cell types and neurons or between different brain layers.
Byron YU, professor of biomedical engineering and electrical and computer engineering at Carnegie Mellon, concludes:
“The introduction of DLAG is like introducing a scalpel to gain potentially deeper insights into how areas of the brain communicate with each other. Along with this paper, we are making our source code available to others in the scientific community. DLAG can be used to study other brain systems outside of the visual system where we have focused, for example to study memory, decision making and motor control.”
Article source:
“Disentangling the flow of signals between populations of neurons.”
Study published August 18, 2022 in Nature Computational Science,
doi.org/10.1038/s43588-022-00282-5
Authors and affiliations:
- Department of Electrical and Computer Engineering, Carnegie Mellon University, Pittsburgh, PA, USA: Evren Gokcen, João D. Semedo and Byron M. Yu;
- Dominick Purpura Department of Neuroscience, Albert Einstein College of Medicine, New York, NY, USA: Anna I. Jasper, Amin Zandvakili and Adam Kohn;
- Department of Ophthalmology and Visual Sciences, Albert Einstein College of Medicine, New York, NY, USA: Adam Kohn;
- Department of Systems Biology and Informatics, Albert Einstein College of Medicine, New York, NY, USA: Adam Kohn;
- Champalimaud Neuroscience Programme, Champalimaud Foundation, Lisbon, Portugal: Christian K. Machens;
- Department of Biomedical Engineering, Carnegie Mellon University, Pittsburgh, PA, USA: Byron M. Yu.
Translated from Neurosciences : comprendre les flux de communication dans le cerveau grâce au machine learning