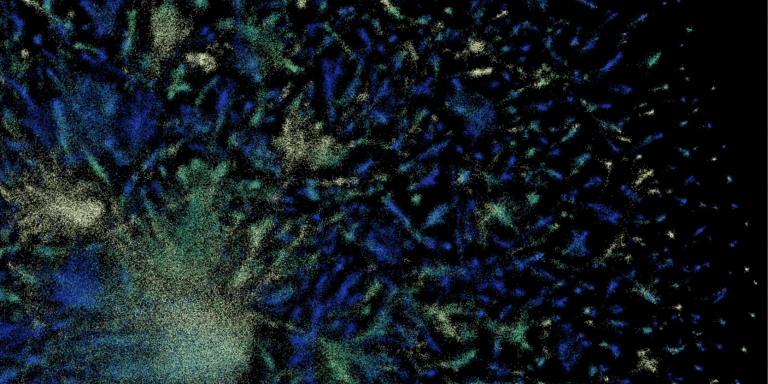
Meta CEO Mark Zuckerberg announced this November 1 that Meta AI has created a model that predicts protein folding 60 times faster than state-of-the-art models: ESM-2, which has 15 billion parameters. The company published it along with the ESM (Evolutionary Scale Modelling) Metagenomic Atlas, a database of 617 million metagenomic protein structures, while the DeepMind-developed AlphaFold 2, which also predicts the three-dimensional structure of a protein based on its amino acid sequence, has “only” 200 million.
Proteins are present in all living cells where they perform essential functions. The rods and cones in our eyes that detect light and allow us to see, the molecular sensors that underlie hearing and our sense of touch, the complex molecular machines that convert sunlight into chemical energy in plants, the enzymes that break down plastic, and the antibodies that protect us from disease are all examples of proteins.
The metagenomic proteins found in microbes including soil, air, at the bottom of the ocean and even inside our gut, are far more numerous than those that make up animal and plant life, but still little understood.
Metagenomics uses genetic sequencing to discover proteins in samples from these complex environments. It has revealed the incredible breadth and diversity of these proteins, discovering billions of novel protein sequences, catalogued in large databases compiled by public initiatives such as NCBI, the European Bioinformatics Institute, and the Joint Genome Institute, integrating studies from a global community of researchers.
According to Meta, ” ESM Metagenomic Atlas, is the first to cover metagenomic proteins in a comprehensive and large-scale manner. These structures provide an unprecedented view of the breadth and diversity of nature, and offer the potential for new scientific insights and accelerated protein discovery for practical applications in fields such as medicine, green chemistry, environmental applications and renewable energy.”
The creation of this “ first ‘dark matter’ overview of the protein universe” was made possible by the development of ESM-2, a model for protein folding from Meta AI.
ESM-2, a protein language model with 15 billion parameters
In 2019, Meta had published a study showing that language models learn properties of proteins, such as their structure and function. Using a form of self-supervised learning known as masked language modeling, the researchers had trained a language model on the sequences of millions of natural proteins. With this approach, the model must correctly fill in the blanks in a passage of text, such as “To __ or not to __, that is the ____.” They trained a language model to fill in the blanks in a protein sequence, such as “GL_KKE_AHY_G” across millions of diverse proteins.
The next year they developed, ESM-1b, a model with about 650 million parameters that is used for a variety of applications, including helping scientists predict the evolution of COVID-19 and discover the genetic causes of the disease.
The researchers extended this approach to create a next-generation protein language model, ESM-2, which, with 15 billion parameters, is the largest protein language model to date. They found that from 8 million parameters, information emerged in internal representations that predicted 3D structure at atomic resolution.
The ESM-2 neural network created the ESM Metagenomic Atlas by predicting 617 million structures from the MGnify90 protein database in just two weeks of operation on a 2,000 GPU cluster. Both are expected to accelerate the discovery of new drugs, help fight disease and develop clean energy.
Translated from Meta présente « ESM Metagenomic Atlas », une base de données de 617 millions de structures protéiques métagénomiques