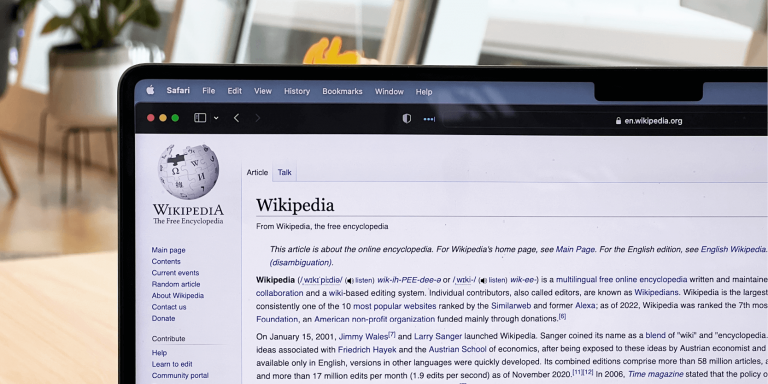
When we do a search on the Internet, the search engine very often suggests the site of the community encyclopedia Wikipedia. It contains about 6.5 million articles by volunteer contributors, but how can we know if these are reliable, even though the sources of the articles are cited? Meta relied on Meta AI’s research and advances to develop SPHERE, an open source model capable of automatically analyzing hundreds of thousands of citations at a time to check whether they actually support the corresponding claims, it recently published it on the Github platform.
Meta said it is not partnering with Wikimedia, the foundation that runs Wikipedia, on this project. Its goal is to create a platform to help Wikipedia editors systematically spot citation problems and quickly correct the citation or the corresponding article content.
Sphere, a retrieval and verification library
In September 2020, Facebook AI had introduced KILT (Knowledge Intensive Language Tasks), an AI model that integrates information retrieval and verification. It brings together 11 datasets created from a pre-processed collection of the entire Wikipedia corpus in a single format, allowing for balanced evaluation across different models with increased accuracy.
Meta AI continues to train neural networks on more nuanced representations of language so that they can identify relevant sources in an Internet-sized data pool. Natural language understanding (NLU) techniques estimate the probability that a claim can be inferred from a source. In NLU, a model translates human sentences (or words and paragraphs) into complex mathematical representations. The tools designed by Meta AI aim to compare these representations to determine whether one claim supports or contradicts another.
A dataset of 134 million Web pages
One of the main components of the Sphere system, a Web-scale retrieval library, is a new 134 million data set, divided into 906 million runs of 100 tokens each.
Meta AI used AI to index a large amount of information and help find the appropriate sources among all that data. For example, the company fed its algorithms with 4 million queries from Wikipedia to train them to focus on a single source from a large set of web pages to validate each statement.
During a search, the models create and compare mathematical representations of the meaning of entire statements rather than individual words. Because Web pages can contain long stretches of text, the models evaluate content in blocks and consider only the most relevant passage when deciding whether to recommend a URL. These predefined indexes, which list 40 times more content than other Wikipedia indexes, will be included in Sphere.
Meta AI says, once deployed in the real world, the model will offer the most relevant URLs as potential citations for a human editor to review and approve. For now, the team continues to refine it, the next step will be to train models to assess the quality of retrieved documents, detect potential contradictions, prioritize more reliable sources.
Translated from Meta AI présente Sphere, un modèle conçu pour vérifier les citations sur Wikipedia