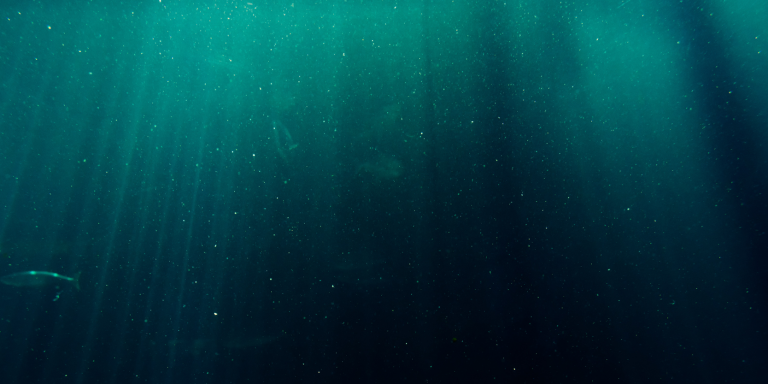
As part of an international collaboration, a team of researchers from the Laboratoire d’Océanographie de Villefranche sur Mer (LOV, Sorbonne University/CNRS) has assembled a huge zooplankton dataset acquired by underwater cameras on a global scale, the analysis of which has allowed the modeling of the composition and oceanic biomass of zooplankton. The results of this study, entitled “Global Distribution of Zooplankton Biomass Estimated by In Situ Imaging and Machine Learning” were published in Frontiers of Marine Sciencein August.
Plankton consists of a wide variety of organisms, ranging from bacteria to vertebrates, protists and algae, living adrift in the aquatic and marine compartments of the globe and playing a central role in marine food chains and geochemical cycles. These taxonomically diverse organisms, ranging in size from a few micrometers to several meters, produce 50% of the carbon dioxide, allow carbon sequestration and are the basis of the marine food chain.
To rapidly quantify their diversity and biomass in the oceans is essential. Satellites, such as Seasat launched in 1978, can observe autotrophic plankton on a global scale, but they can only do so in the first few meters of the surface. Expedition ships, on the other hand, can obtain data at depth but locally. In addition, the processing of samples brought back on board is often long and tedious. The global biomass of zooplankton is thus very poorly known.
The study
For this study, the team collected a huge data set on zooplankton acquired by underwater cameras at the global scale. Its analysis, done in the framework of Laetitia Drago’s current PhD thesis at LOV, allowed to model the composition and the oceanic biomass of zooplankton.
The study predicted, for the first time, the global biomass distribution of 19 zooplankton taxa ranging from 1 to 50 mm in spherical diameter, using observations made with the Underwater Vision Profiler 5, an in situ quantitative imaging instrument. After classifying 466,872 organisms from more than 3,549 profiles (0-500 m) obtained between 2008 and 2019 worldwide, the researchers estimated their individual biovolumes and converted them to biomass using taxon-specific conversion factors.
They then combined these biomass estimates with climatologies of environmental variables (temperature, salinity, oxygen …), to build habitat models using boosted regression trees.
Results of the study
The results reveal maximum values of zooplankton biomass around 60° N and 55° S as well as minimum values around the major rotating ocean currents, the oceanic gyres, particularly in the southern hemisphere.
The lowest biomass values were predicted north of 80°N and in the Weddell Sea, the researchers also observed an increase in predicted biomass around the equator. The highest biomass values were predicted between 50 and 80°N, in the coastal waters of the Labrador Sea and Baffin Bay, and in the Greenland Sea. Relatively high biomass was predicted around these locations as well as in the Gulf of Alaska, Bering Sea and Sea of Okhotsk. A band of high biomass was predicted between 40 and 50°S, a region associated with the Arctic Front.
The overall integrated biomass (0-500 m) was estimated at 0.403 PgC. It was largely dominated by Copepoda (35.7%, mainly in polar regions), followed by Eumalacostraca (26.6%) Rhizaria (16.4%, mainly in the intertropical convergence zone).
The machine learning approach used here is sensitive to the size of the training set and generates reliable predictions for abundant groups such as Copepoda (R2 ≈ 20-66%) but not for rare groups (Ctenophora, Cnidaria, R2 < 5%). Nevertheless, this study provides a first protocol for estimating global spatially resolved zooplankton biomass and community composition from in situ imaging observations of individual organisms.
The underlying dataset covers a 10-year period, whereas approaches that rely on net samples use datasets collected since the 1960s. Increased use of digital imaging approaches should allow estimates of zooplankton biomass distribution at the basin scale to be obtained on a global scale in shorter time frames in the future.
Article Sources:
” Global Distribution of Zooplankton Biomass Estimated by In Situ Imaging and Machine Learning.”
Authors:
- Laetitia Drago, Thelma Panaïotis, Jean-Olivier Irisson, Lionel Guidi, Marc Picheral, Lars Stemman and Rainer Kiko from Sorbonne University, Laboratoire d’Océanographie de Villefranche-sur-mer;
- Marcel Babin of the Takuvik International Research Laboratory, Ocean Quebec, Laval University (Canada) – Centre national de la recherche scientifique (CNRS), Department of Biology and Quebec-Ocean, Laval University, QC, Canada;
- Tristan Biard of the Laboratoire d’Océanologie et de Géosciences (LOG), Univ. Littoral Côte d’Opale, Univ. Lille, Centre National de la Recherche Scientifique (CNRS), UMR 8187, Wimereux, France;
- François Carlotti of the Département Ecologie Marine et BIOdiversité (EMBIO), M.I.O. Institut Méditerranéen d’Océanologie Bâtiment Méditerranée, Marseille, and of the Laboratoire d’Océanographie Physique et Biologique (LOPB), case 901 13288, Marseille;
- Laurent Coppola of Sorbonne University, Laboratoire d’Océanographie de Villefranche-sur-mer and Sorbonne University, Centre National de la Recherche Scientifique (CNRS), OSU STAMAR, Paris;
- Leep Karp-Boss of the School of Marine Sciences, University of Maine, Orono, ME, USA;
- Fabien Lombard of Sorbonne University, Laboratoire d’Océanographie de Villefranche-sur-mer, and the Institut Universitaire de France (UITA), Paris;
- Andrew M. P. McDonnell of the Department of Oceanography, University of Alaska Fairbanks, Fairbanks, AK, USA;
- Andreas Rogge of Section Benthopelagic Processes, Alfred Wegener Institute Helmholtz Center for Polar and Marine Research, Bremerhaven, Germany;
- Anya Mary Waite of the Ocean Frontier Institute and Oceanography Department, Dalhousie University, Halifax, NS, Canada;
- Helena Hauss of the Department of Ocean Ecosystem Biology, GEOMAR Helmholtz Centre for Ocean Research Kiel, Kiel, Germany;
Frontiers of Marine Science, 09 August 2022 Sec. Ocean Observation
DOI: 10.3389/fmars.2022.894372
Translated from Le machine learning et des caméras sous-marines pour prédire la répartition mondiale du zooplancton