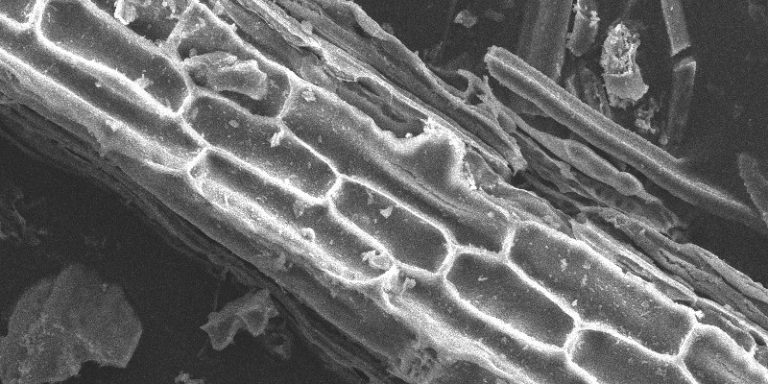
A research team consisting of experts from Pohang University of Technology (POSTECH) and the Korea Institute of Materials Science (KIMS) have developed a technique that can detect and improve the quality of unattended scanning electron microscopy (SEM) images. To do so, they exploited artificial intelligence and more specifically deep learning and applied it to the SEM system. This research project waspublished in a paperwith Seungchul Lee as the lead author.
The importance of quality in scanning electron microscopy
Juwon Na, Gyuwon Kim, Seong-Hoon Kang and Se-Jong Kim accompanied Seungchul Lee in the writing of this article highlighting the use of deep learing for scanning electron microscopy. Scanning electron microscopy is one of the most powerful material analysis equipment and is frequently used to study the relationship between chemical, physical and mechanical properties of materials by providing their microstructural image data.
However, obtaining quality images requires the operator to be highly skilled. All of his manipulations must be of great precision in order to maneuver the system correctly. If this condition is not met, the microscopic images may be of poor quality. Clear and accurate images are essential, as they directly affect the material analysis process.
To solve this problem, several researchers from POSTECH and KIMS have developed a method based on artificial intelligence. They received support from the Mid-Career Research Program, the Priority Research Centers Program of the National Research Foundation of Korea, and the AI Graduate Program of the Institute for Information & Communication Technology Promotion (IITP).
A deep learning method to improve image quality
The research team developed a deep learning-based refocusing method that aims to automatically detect and improve the quality of SEM images. This technology is based on a multi-scale deep neural network and has shown that image quality can be improved by taking into account blind parameters without any knowledge or assumption about the degree of image degradation or blurring.
In addition, the researchers developed a technique to train the network to learn the method as well as the positioning of the refocusing in non-uniformly defocused images.Professor Seungchul Lee said of the research he is leading:
“We expect that the cost and time of developing new materials will be reduced by automating the SEM imaging process of scanning electron microscopy, which is widely used for the research and development of new materials.”
Translated from Des chercheurs coréens exploitent le deep learning pour améliorer la microscopie électronique à balayage