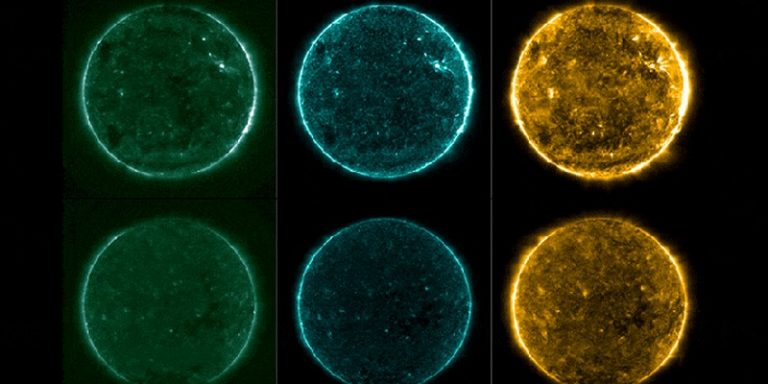
Since 2010, NASA’s Solar Dynamics Observatory (SDO) has been actively working to provide high definition images of the sun. To achieve this, they have been operating a solar telescope for more than a decade whose lenses and sensors are gradually degrading as scientists use it. To extend the life of this instrument, a group of researchers has harnessed AI to help the telescope calibrate itself and provide quality images of the brightest star closest to Earth.
Solar telescopes need regular calibration to work properly
As we all know, it is totally impossible to look at the sun with our eyes without being hurt by it. Fortunately, solar telescopes can perform this task much more accurately than any other tool. The Atmospheric Imaging Assembly (AIA) is one of two imaging instruments in the SDO. The tool constantly looks at the Sun, taking images at 10 wavelengths of ultraviolet light every 12 seconds.
However, over time, the sensitive lenses and sensors that make up this instrument tend to degrade and return images that are of lower quality than expected. To solve this problem and to ensure that the data returned by these instruments are always of the highest quality, scientists recalibrate these instruments from time to time to compensate for “changes” in the instrument due to the gradual degradation of its components.
In order to perform this calibration, scientists use sounding rockets that make short flights into space and carry everything necessary to complete the process. All of the instruments that the sounding rocket carries can see the ultraviolet wavelengths that are measured by AIA. However, there are some drawbacks to this technique: the sounding rockets must be launched at specific times and cannot be operated whenever the scientists want.
Artificial intelligence and machine learning to perform a virtual and permanent calibration
In order to carry out this calibration virtually and thus circumvent the drawbacks of the sounding rocket technique, researchers decided to exploit machine learning. All of their results were reported in a paper by Luiz FG Dos Santos, Souvik Bose, Valentina Salvatelli, Brad Neuberg, Mark CM Cheung, Miho Janvier, Meng Jin, Yarin Gal, Paul Boerner and Atılım Güneş Baydin.
The research team trained a machine learning algorithm so that it could recognize solar structures and compare them using AIA data. They trained the model using images from sounding rocket calibration flights and to test it, they provided it with similar images to see if they were successful in determining which calibration was needed.
With this new process, scientists can continuously calibrate the AI images between sounding rocket flights, improving the accuracy of the images for the ROS experts.
Translated from Comment la NASA exploite le machine learning pour calibrer ses télescopes et obtenir des images de qualité ?