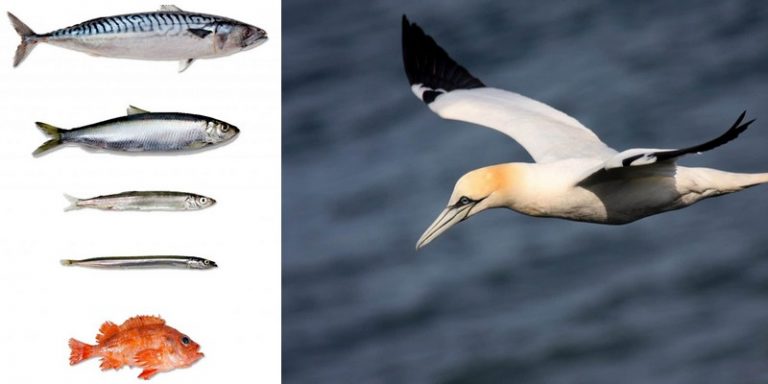
Quebec researchers have harnessed artificial intelligence, specifically deep learning, to better understand the diet of the Northern Gannet, a seabird native to the North Atlantic. They connected a miniature camera, a GPS and a dive recorder to some of the animals in Bonaventure Island and Rocky Mountain National Park. These three tools allowed them to obtain precise information on the marine animals.
A project to better understand the marine behaviour and diet of gannets
How can we speed up the detection and identification of the fish that appear in the astronomical quantities of video images captured by the cameras attached to gannets? This is the central problem of the research project by David Pelletier, researcher and teacher at the Cégep de Rimouski, Pauline Martigny, doctoral student at the same Cégep, Magella Guillemette, researcher at the Université du Québec à Rimouski, and Olivier Leclerc, computer scientist at the Centre de développement et de recherche en intelligence numérique (CDRIN) in Matane.
Their project is called “Digital Gannet” and its objective is to capture gannets, Europe’s largest seabird, and then attach a miniature camera, a GPS and a dive recorder to these animals. All of this equipment weighs a maximum of 75 grams. Each has a task to perform:
- The GPS is placed on the bird’s tail. It records the bird’s position every 10 minutes for two weeks to see if it is flying, resting or fishing.
- The recorder or dive recorder is attached to the numbered metal ring that is fastened around the animal’s leg. The device measures changes in hydrostatic pressure and water temperature several times per second to better understand the bird’s ecological niche.
- The camera is attached to the lower back of the gannet. Custom-designed for the project, the camera only activates and records images when the bird is in the water or when the camera is wet, thus avoiding unnecessary recordings and maximizing battery capacity and recording storage.
After installing these devices, the researchers release the birds, leave them in captivity for 24 hours and then recapture them to remove the devices, which will then be placed on other individuals. With this method, the researchers hope to follow about 60 gannets with their five cameras. With eight hours of recordings per camera and sixty birds, that’s 480 hours of potential film to analyze. This is a huge amount of video that will have to be analysed, and this is where artificial intelligence comes into play.
The role of artificial intelligence in this project
Working with Olivier Leclerc, the researchers were able to develop an artificial neural network capable of recognizing five species of fish eaten by gannets: mackerel, herring, capelin, sand lance and redfish. This neural network first had to be trained to enable the computer to recognize fish in images. This training was done by presenting the algorithm with thousands of images first labelled by an expert, who had previously indicated the elements corresponding to the fish to be recognised and the species to which it belongs.
Isabelle Cayer, executive director of CDRIN, gives more details on how the deep learning model works:
“The person annotating must be an expert who knows how to recognize the distinctive characteristics of species. [The machine must learn, for example, that the fin of one species is sharper than that of another. For example, the machine will mathematically discover that mackerel with black and blue stripes on their backs have alternative patterns of black and blue: the algorithm creates analysis rules like that for itself, which it will then apply in other photos.”
To retrieve all the data needed to train the neural network, David Pelletier installed cameras in aquariums at the Exploramer museum in Sainte-Anne-des-Monts, obtained data sets on redfish in the Maurice Lamontagne Institute’s tanks and photos of mackerel from fishermen on the docks. He says that his network is accurate eight times out of ten at the moment and that the task for him is not easy because the fish move quickly and when the bird dives, it creates broth, a multitude of small air bubbles that the tool can interpret as fish.
With these three devices and artificial intelligence to analyze all of the data collected, the research team hopes to improve their model to fill in some of the gaps in studying lesser-known fish.
Translated from Comment le deep learning et les réseaux de neurones sont utilisés pour analyser des données de biologie marine ?