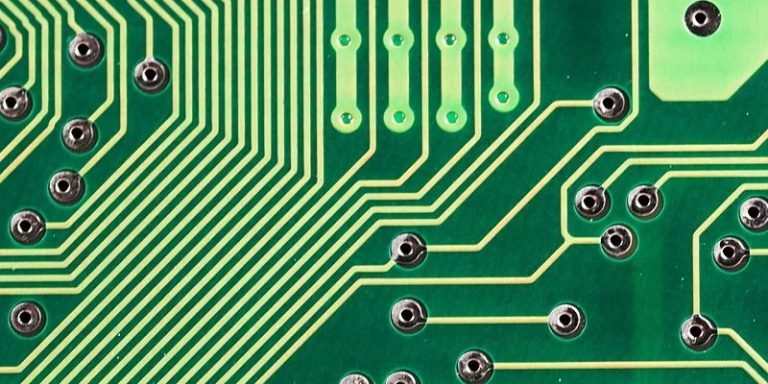
Last May, Google announced in its 2021 edition of Google I/O, the fourth generation of its TPU chips (TPUv4). A research team from the Mountain View firm has developed a machine learning model, and more precisely, reinforcement learning, capable of designing the components of a computer chip. This system could make it possible, like competing solutions , to design these microprocessors in a few hours instead of taking several weeks or months.
Chip manufacturing: a process that can take several months
In order to design the architecture of computer chips, several particularly time-consuming steps follow one another, taking several weeks or even months. In an effort to optimize chip design time, a team of Google researchers have designed a machine learning model that, according to them, allows a design plan for the components of a chip to be put in place in less than six hours.
All the details about this machine learning model are available in the article published on June 9 and whose main authors are Azalia Mirhoseini and Anna Goldie. According to the experts who worked on this system, this method automatically generates chip floor plans that are superior or comparable to those produced by humans in all key metrics, including power consumption, chip size and performance.
A Reinforcement Learning Model
Computer chips are typically composed of dozens of individual modules ranging from memory subsystems to computing units. Their planning involves placing these components on a two-dimensional grid while ensuring that they are properly linked. This process is gradually becoming very complex to implement on modern chips. Human designers taking several months and up to 72 hours to evaluate the placement of a part.
To automate this process, the researchers exploited reinforcement learning: they created a dataset based on 10,000 different designs that serves as an anchor point. Subsequently, the model will learn by doing by adjusting the parameters according to the situation. The neural network thus designed can evaluate a variety of individual modules as well as their location. The research team states in their paper:
“In our experiments, we show how our agent is exposed to a greater volume and variety of chips. It becomes both faster and more efficient at generating optimized locations for new chip blocks, bringing us closer to a future in which chip designers are assisted. by artificial agents with extensive experience in chip placement.”
Towards a reduction in chip design time
In addition to significantly speeding up chip planning, the new approach could allow microprocessor designs to be optimized at earlier stages of the chip design process for better performance. In addition, it could also enable optimization of chip architectures at the macro scale, and potentially even facilitate full automation of the chip design process.
The reduction in chip design time is a breakthrough that would be welcomed by the entire supply chain. In addition, the multinational technology company has ambitions to design custom processors, particularly in the form of system-on-chip.
Companies such as Samsung have already brought in experts in the field to help reduce the production time of these electronic components, which are currently in the greatest shortage in history. There is no doubt that this reinforcement learning model could be another step towards the realization of this project.
Translated from Google élabore un modèle d’apprentissage par renforcement pour fabriquer des puces plus rapidement