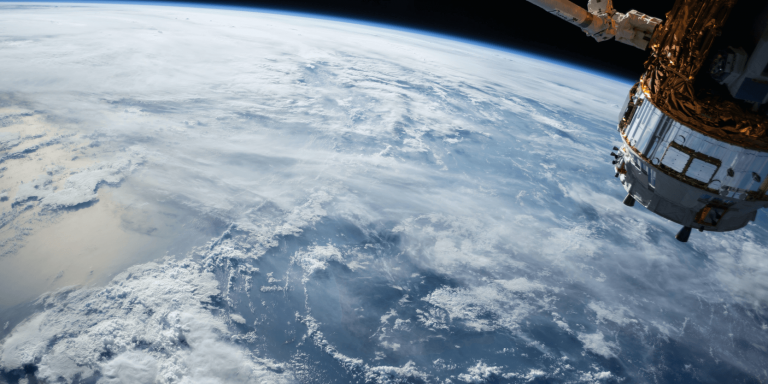
The Intelligence Advanced Research Projects Activity (IARPA), the research arm of the U.S. intelligence community, has launched the Space-based Machine Automated Recognition Technique (SMART) program to detect, monitor and characterize large-scale construction projects and other man-made or natural activities across the planet, such as crop growth.
There are currently more than 150 earth observation satellites in orbit, and the images they provide are the best sources of data for understanding the evolution of the earth’s ecosystems. However, these satellites have different characteristics, the data are numerous, varied and cannot be exploited manually, the goal of SMART is to harmonize them.
In announcing the project, IARPA officials said:
“The volume of geospatial intelligence continues to grow, while analysts struggle with the volume, variety, and velocity of spatial data to support local, regional, and national decision making. SMART seeks to bridge a gap in expertise, infrastructure, and data preparation by developing easy-to-implement tools for analysts to effectively analyze, evaluate, and use megadata.”
The SMART program
Announced in 2019, the SMART program began in 2021, will run in three phases, and is planned for 4 years. Initially, it focused on heavy construction detection and monitoring, and the transferability of the approach to other forms of natural and anthropogenic change will be explored later.
Detection of construction sites
Large scale construction typically takes years, it can be detected from changes in terrain, dump trucks or cranes, however these may not be visible at the same time or may be visible in contexts other than construction, so many objects and changes in terrain over time need to be identified and a model derived.
From January 2021 to April 2022, during the 1st phase, competitors built models that took into account features that were present in the same place but not at the same time, regardless of the source of the image.
Jack Cooper, program director, explains:
“It’s how all these puzzle pieces fit together over time that defines the heavy construction. And that’s what makes it challenging. It’s an activity. It’s not just one object, not just one change.”
The data used
IARPA provided 100,000 satellite images of 27 regions from Dubai to pristine areas of the Amazon rainforest, covering about 90,000 square kilometers in area, taken between the years 2014 and 2021. They came from four sets of satellites: from Landsat, a program shared by NASA and the United States Geological Survey, the European Space Agency’s Sentinel, and Maxar and Planet.
The selected models
The models had to identify large-scale construction areas, classify the stage of construction and alert analysts to specific changes. They also had to be able to identify certain characteristics such as thermal anomalies, soil permeability and types of equipment present.
Last June, AFS, BlackSky, Kitware, Systems & Technology Research, Applied Research Associates and Intelligent Automation were selected for the second phase, which will last 18 months.
Kitware engineers used a combination of methods to analyze the pixels, including hardware characterization and semantic segmentation, involving a transformer, while AFS opted for unsupervised learning.
The next phases of the program
In the second phase, which will last until 2023, the teams will adapt their construct recognition models to different time-changing tasks such as crop growth detection.
The third phase, beginning in 2024, will challenge participants to build systems that generalize to different types of land use.
There won’t necessarily be a single winner; IARPA’s goal is to transfer promising technology to intelligence agencies that can use it in the real world.
Jack Cooper states:
“IARPA makes phase decisions based on performance against our metrics, diversity of approaches, available funding, and analysis of our independent testing and evaluation. At the end of Phase 3, there may be no teams or more than one team remaining. The best solution might even combine pieces from multiple teams…”
Translated from Focus sur SMART, programme de l’IARPA visant à identifier et surveiller les constructions à grande échelle grâce au machine learning