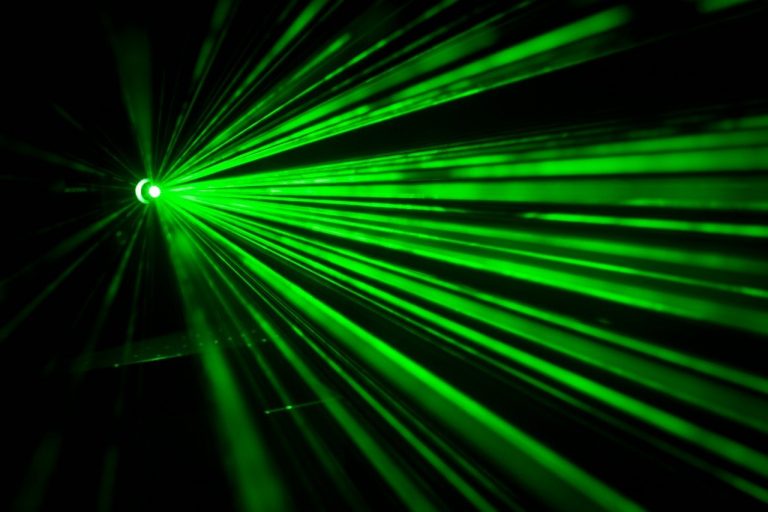
In a close international collaboration, researchers from the FEMTO-ST institute, attached to the CNRS and the University of Bourgogne Franche-Comté, have recently published their synthesis of the latest advances in the field of ultrafast photonics. Lasers and ultrafast photonic devices are complex dynamic systems that are difficult to model. Artificial intelligence based on machine learning techniques is a promising way to accelerate and improve their design.
Ultrafast lasers, which deliver ultrashort pulses ranging from a few picoseconds to a few femtoseconds (between 10-12 and 10-15 seconds) for the fastest ones, are used in particular in telecommunications, biological imaging, industry, and medicine. The design of these complex and non-linear photonic systems is mostly based on numerical simulations and tests that lead to performance compromises or limitations.
To overcome these limitations, while photonics applications require stable and perfectly adapted performances, several research teams in the world are exploring a new way, based on artificial intelligence techniques, more precisely on machine learning. In this context, an international group of scientists, including FEMTO-ST researchers, has published a first review article in the journal Nature Photonics which provides a comprehensive overview of how machine learning can revolutionize the development of laser sources and ultrafast photonic systems.
The review article, written by the international team, first shows how machine learning algorithms (genetic algorithms) have been used to design ultrafast laser sources whose characteristics are determined according to the desired mode of operation. Secondly, the paper focuses on other algorithms -neural networks- that facilitate the shaping of the pulse (compression, generation of arbitrary pulse shapes) at the output of the laser cavity to suit the application. Finally, the researchers highlight machine learning techniques that also make it possible to analyze physical phenomena in order to identify key parameters, thus constituting an aid to the construction of models.
The operation of an ultrafast photonic device depends on a large number of parameters, which makes it difficult to simulate from a physical model. Machine learning techniques, which directly identify consistent patterns in large amounts of data, allow the automatic extraction of parameter sets that produce the desired modes of operation and performance. Studies in these areas are in full development and, according to the authors, should now explore other machine learning techniques, as well as the combination of several techniques used separately so far.
In a second article for the journal Nature Machine Intelligence, the FEMTO-ST team, in collaboration with the University of Tampere (Finland), also reports on their very recent work. Their research shows how a recurrent neural network can predict the dynamic behavior of ultrashort laser pulses in an optical fiber. This method makes it possible to optimize the propagation of the pulse for the application. The study demonstrated the effectiveness of machine learning techniques applied to pulse compression, and supercontinuum generation (a very broad spectrum laser source). Here again, the help of artificial intelligence avoids the need to solve complex mathematical models, requiring sometimes prohibitive computing resources.
The publications of the FEMTO-ST researchers can be consulted:
Here for the article published on Nature Photonics
Here for the article published on Nature Machine Intelligence
Translated from L’Institut FEMTO-ST met l’intelligence artificielle au service de ses recherches en photonique ultrarapide