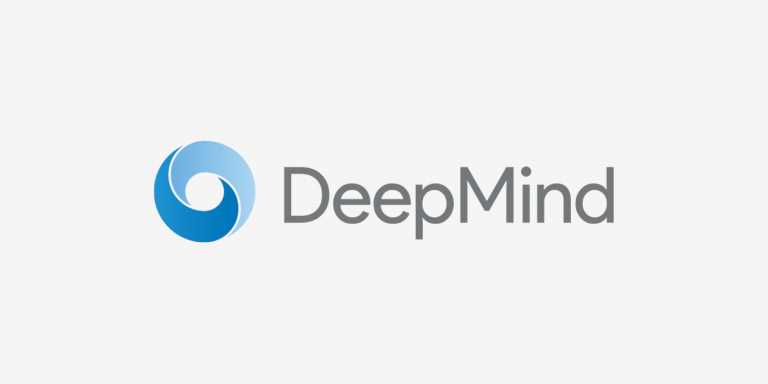
For AIs, board games are great learning grounds, which has allowed them to beat humans at Go, chess, poker, backgammon… Stratego is one of the board games that AI has yet to master, researchers at Deepmind AI present “DeepNash,” an autonomous agent trained with model-free multi-agent reinforcement learning that learns to play Stratego at expert level.
For years, the AI research community has been interested in the board game Stratego, created in 1947, which has since gone through several versions. This game of strategy and bluffing is played by two players, each of whom tries to take the other’s flag. It is very complex: if each player has 40 pieces of different values, he doesn’t know where the enemy’s flag is or which pieces are in front of him, so he doesn’t know their value. The game board has 100 squares, eight of which are occupied by two impassable lakes. The players start the game by placing their pieces on the first four lines of the board in front of them (phase 1).
The second phase of the game begins, the players take turns moving pieces, except for the flag and the six bombs which are fixed pieces. When two pieces meet, their value is revealed and the weaker piece is removed (or both if they have the same strength). When the weaker moving piece, the Spy, attacks the Marshal, of value 10, he wins however and the 10 is captured.
While it can be likened to a mix of poker and chess, unlike the latter, reinforcement learning (RL) algorithms did not meet the expectations of AI researchers and did not really address the two main challenges of this game, namely the10535 potential states in the Stratego game tree and the 1066 possible deployments at the beginning of the game. Indeed, existing AI methods barely reach an amateur level of play.
Deepnash
Developing intelligent agents that learn end-to-end to make optimal decisions under imperfect information in Stratego, from scratch, with no demonstration data, is therefore the challenge Deepmind AI researchers set themselves. To do so, they chose the most complex version of the game: Stratego Classic and introduced Deepnash, an autonomous agent that beat the best existing methods and reached the level of an expert game.
DeepNash relies on a principled, model-free RL algorithm called Regularized Nash Dynamics (R-NaD) combined with a deep neural network architecture to converge to an epsilon-Nash equilibrium (The notion of Nash Dynamics refers to mathematician John Forbes Nash in game theory).
A Nash equilibrium guarantees that the agent will work well, regardless of the opponent. It is often used to play against humans in two-player zero-sum games.
The Regularized Nash Dynamics (R-NaD) algorithm, based on the concept of regularization, implemented via the deep neural network, thus converges to an approximate Nash equilibrium, instead of “cycling” around it, and directly modifies the underlying multi-agent learning dynamics. (figure 1b)
Evaluation of Deepnash
The team then evaluated Deepnash’s performance on the Gravon platform, an online game server well known to Stratego players where it was measured against eight AI bots. The table below shows its efficiency as it won 97% of the challenges:
It was also tested for two weeks last April against the best human players and won 84% of the 50 games, placing it in 3rd place in the Stratego 2022 Classic challenge. Deepnash has thus demonstrated its capabilities for deployment, bluffing and compromise.
DeepNash could unlock further RL applications for real-world multi-agent problems characterized by imperfect information that are currently beyond the reach of state-of-the-art AI methods.
Article source:
ArXiv: “Mastering the Game of Stratego with Model-Free Multiagent Reinforcement Learning” (arXiv:2206.15378)
Julien Perolat , Bart de Vylder , Daniel Hennes , Eugene Tarassov , Florian Strub , Vincent de Boer , Paul Muller , Jerome T. Connor , Neil Burch , Thomas Anthony , Stephen McAleer , Romuald Elie , Sarah H. Cen , Zhe Wang , Audrunas Gruslys , Aleksandra Malysheva , Mina Khan , Sherjil Ozair , Finbarr Timbers , Toby Pohlen , Tom Eccles , Mark Rowland , Marc Lanctot , Jean-Baptiste Lespiau , Bilal Piot , Shayegan Omidshafiei , Edward Lockhart, Laurent Sifre , Nathalie Beauguerlange , Rémi Munos , David Silver , Satinder Singh , Demis Hassabis , Karl Tuyls.
Translated from Deepmind AI présente « DeepNash », l’agent autonome RL sans modèle, expert du jeu « Classic Stratego »