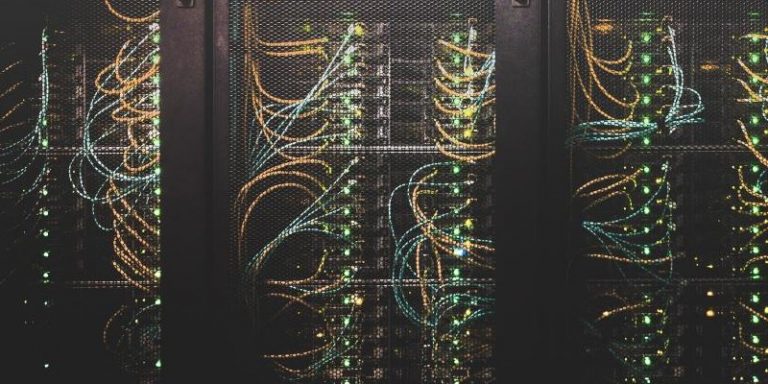
In recent years, efforts have been made to reduce the energy demand of AI systems, which has led to a restructuring of the methods used. However, a team of researchers has just discovered that this structure can be attacked in order to make the machine work much harder and consequently perform many more calculations. A cyber attack of this type could cause power consumption to skyrocket and thus, be the opposite of what the model originally proposed.
A model for reducing the power consumption of AI systems
The enormous power consumption of large AI models has led researchers to design more efficient neural networks. One of the proposed solutions is to use adaptive multi-agent systems that work by dividing the tasks to be performed in relation to their difficulty of resolution. Then, the tool spends the least amount of resources possible to perform each task.
Let’s take the example of two photographs: one showing a man standing in a totally clear landscape and the other with the same man sitting in a much more complex landscape. A conventional neural network would run both photos through all its layers and spend the same amount of computing power to label each of the two images. But the adaptive multi-agent system will make the easier-to-label picture (the first one) go through only one or two layers, which is quite necessary to complete the task.
This reduces the carbon footprint of the AI model, also improves its speed and allows it to be deployed in small devices such as smartphones and smart speakers. But this type of neural network means that if we change the input, i.e. the image it receives, we could change the amount of computation we would need to solve it, making the system vulnerable.
A cyber attack on deep neural networks
Similar to a denial of service (DDoS) attack, this cyber attack seeks to clog a network to make it unusable. The model’s deep neural network is forced to use far more computational resources than necessary in order to slow down its “thinking” process. Sanghyun Hong, Yiğitcan Kaya, Ionuţ-Vlad Modoranu, and Tudor Dumitraş, researchers at the University of Maryland for the Maryland Cybersecurity Center, noticed this vulnerability as part of their study of deep neural networks (DNNs) and the attacks they can face.
In one experiment, they generated interference at the input of a DNN which forced the system to increase its computing power needed to complete a task. Even though the person hacking the model had limited information about it, the researchers deduced that it was still able to slow down network processing and increase power consumption by 20 to 80%. This is due to the fact that attacks can be easily transferred via different types of neural networks.
However, this kind of attack is still quite theoretical and these studies serve as a cautionary tale. Adaptive multi-agent systems are bound to be used more frequently especially in the IoT.
Translated from Une cyberattaque pourrait bien faire grimper la consommation d’électricité des systèmes d’intelligence artificielle