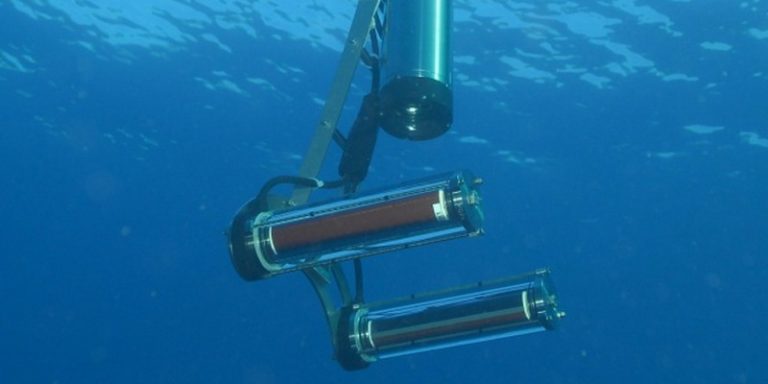
As part of a mission, several researchers captured in situ images of the planktonic spring bloom in the Arctic Ocean, resulting in a huge database. A team from the Laboratoire d’océanographie de Villefranche (LOV) at Sorbonne University and the Takuvik International Research Laboratory at Laval University in Canada have developed a method to characterize and categorize these millions of images of plankton and marine snow particles. Their solution exploits artificial intelligence and more specifically a convolutional neural network to operate.
The challenges of marine observation
Marine observation is a difficult process to set up, as the marine environment imposes heavy technical constraints such as corrosion and pressure, not to mention its immensity: pelagic ecosystems (relative to the open sea) occupy the largest living space on our planet while participating in the major climatic and biogeochemical balances. The period 2020-2030 is considered by UNESCO’s Intergovernmental Oceanographic Commission (IOC) as “the decade of the oceans” and it particularly supports observation efforts.
Particles and aggregates derived from plankton activity at the surface are also very complex to observe, as they are fragile: this is what is known as “marine snow”. During the last 20 years, underwater cameras were designed by a team from the Laboratoire d’Océanographie de Villefranche to obtain information on each of the objects potentially present in the water.
With these cameras, about ten million images of plankton and marine snow have been taken and recorded. Now that a database has been formed, it is important to identify and sort them in order to understand the dynamics of biological production at the ocean surface. In the future, the cameras will contribute to a massive observation of the oceans, which will increase the flow of images and information to be processed.
The images needed to create the new method were acquired by the camera developed at LOV during the plankton bloom period in the Arctic pack ice at Baffin Bay and in Fram Strait between 0 and 1000m depth.
Creation of a characterization and categorization method using a convolutional neural network
Two articles published last January and May describe a method capable of characterizing and categorizing image banks of marine snow and plankton:
- The first paper entitled Trait-based approach using in situ copepod images reveals contrasting ecological patterns across an Arctic ice melt zone was written by Laure Vilgrain, Marc Picheral, Jean-Olivier Irisson and Sakina-Dorothée Ayata, from the Laboratoire d’océanographie de Villefranche, and Frédéric Maps, Marcel Babin and Cyril Aubry, from the Laboratoire de Recherche International Takuvik.
- The second paper is entitled Marine snow morphology illuminates the evolution of phytoplankton blooms and determines their subsequent vertical export and was written by Emilia Trundnowska, Polish Oceanographic Institute, Léo Lacour and Marcel Babin, International Takuvik Research Laboratory, Andreas Rogge, Alfred Wegener Institute for Polar and Marine Research in Bremerhaven, Germany, Anya M. Waite, from the Department of Oceanography at Dalhousie University, and Mathieu Ardyna and Lars Stemmann, from the Laboratoire d’océanographique de Villefranche.
In these two articles, we find this solution that allows :
- Synthesize the visual aspect of each of the images collected to access the individual characteristics of the planktonic organisms
- Objectively categorize images of marine snow that present a continuum of appearance.
The acquired images were classified into biological groups using a convolutional neural network while validating this classification by taxonomy experts, allowing the separation of images of different plankton organisms from those of marine snow. Morphological descriptors measured on each object were used to determine the main visual characteristics of the plankton and marine snow images.
With this method, researchers were able to associate additional information with the organisms and marine snow to understand the functioning of the marine ecosystem. This solution could be used for many applications such as accurately estimating the vertical fluxes of biogenic particles in the first few kilometres of the oceans, which have a potential impact on climate regulation. However, the researchers are aware that the characterization and categorization of underwater images still needs to be perfected.
Translated from Les réseaux de neurones convolutifs pour catégoriser et classifier les images des ecosystèmes pélagiques