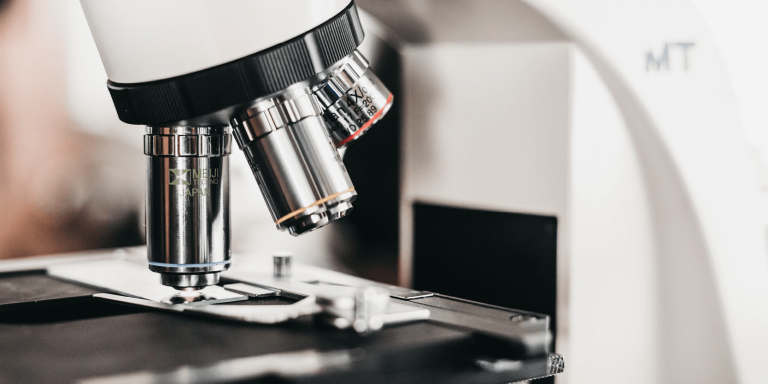
While cancer is one of the leading causes of death in the world (approximately 1 in 6 deaths), early diagnosis increases the chances of a cure. Researchers from the Koch Institute for Integrative Cancer Research at MIT and Massachusetts General Hospital (MGH) used deep learningand built a developmental multilayer perceptron (D-MLP) classifier to identify the origin of cancer. Their study, “Developmental Deconvolution for Classification of Cancer Origin,” was published in late August by Cancer Discovery.
Unknown Primary Cancer (UPC) is cancer that has already spread to other organs in the body (metastasis), but doctors have not found the original tumor. It is usually small, but very aggressive, so oncologists must quickly implement non-targeted treatments that are often toxic for the patient.
This new deep learning-based approach could help classify unknown primary cancers by taking a closer look at gene expression programs related to early cell development and differentiation.
Salil Garg, Charles W. (1955) and Jennifer C. Johnson Clinical Investigator at the Koch Institute and pathologist at MGH, lead author of the study, explains:
“Sometimes you can apply all the tools that pathologists have to offer, and you’re still left with no answers. Machine learning tools like this could allow oncologists to choose more effective treatments and give more advice to their patients.”
A study based on gene expression and deep learning
Cancer cells look and behave very differently from normal cells, in part because of significant alterations in the way their genes are expressed. Advances in single-cell profiling and efforts to catalog different cell expression patterns in cellular atlases, have provided a wealth of data containing clues about the origin of different cancers. Deep learning is an ideal technology to exploit this data.
To make their model more efficient, the researchers had to reduce the number of features while extracting the most relevant information, and focused the model on signs of altered developmental pathways in cancer cells.
During embryo development, undifferentiated cells specialize in various organs, with many pathways guiding how cells divide, grow, change shape and migrate. As the tumor grows, cancer cells lose many of the specialized features of a mature cell. They can be compared to embryonic cells in some respects, as they have the ability to proliferate, transform and metastasize.
The researchers compared two large cell atlases, identifying correlations between tumor and embryonic cells:
- The Cancer Genome Atlas (TCGA), which contains gene expression data for 33 tumor types;
- The Mouse Organogenesis Cell Atlas (MOCA), which describes 56 distinct trajectories of embryonic cells as they develop and differentiate.
MIT postdoctoral fellow Enrico Moiso, also lead author of the study, explains:
“Single-cell resolution tools have radically changed the way we study cancer biology, but how we make this revolution impactful for patients is another matter. With the emergence of developmental cellular atlases, particularly those that focus on early phases of organogenesis such as MOCA, we can extend our tools beyond histological and genomic information and open the door to new ways to profile and identify tumors and develop new treatments.”
The researchers broke down the gene expression of TCGA tumor samples into individual components corresponding to a specific time point in a developmental trajectory and assigned each a mathematical value.
They then built a deep learning model, a developmental multilayer perceptron (D-MLP), that scores a tumor for its developmental components and then predicts its origin.
Tumor classification
After training, D-MLP was applied to 52 new cancer samples from the most challenging cases encountered at MGH from 2017 to 2020 that could not be diagnosed. The model classified the tumors into four categories and provided predictions and other information that could guide the diagnosis and treatment of these patients.
One of the 52 samples was from a patient with a history of breast cancer who had evidence of aggressive cancer in the fluid spaces around the abdomen. D-MLP strongly predicted ovarian cancer, and indeed, a mass was found in the ovary six months later that caused the cancer.
The results of this study provided an atlas of the origins of tumor development, a tool for diagnostic pathology, and suggest that developmental classification may be a useful approach to patient tumors.
For future work, the researchers plan to increase the predictive power of their model by adding other types of data, including information collected from radiology, microscopy, and other tumor imaging.
Salil Garg concludes:
“Developmental gene expression is only a small part of all the factors that could be used to diagnose and treat cancers The integration of radiology, pathology and gene expression information is the real next step in personalized medicine for cancer patients.”
Article Sources:
- Enrico Moiso, Koch Institute for Integrative Cancer Research, Massachusetts Institute of Technology, Cambridge MA and Broad Institute of Harvard-MIT, Cambridge MA;
- Alexander Farahani, Department of Pathology, Massachusetts General Hospital, Harvard Medical School, Boston MA;
- Hetal D. Marble, Department of Pathology, Massachusetts General Hospital, Harvard Medical School, Boston MA;
- Austin Hendricks,Koch Institute for Integrative Cancer Research, Massachusetts Institute of Technology, Cambridge MA;
- Samuel Mildrum, Koch Institute for Integrative Cancer Research, Massachusetts Institute of Technology, Cambridge MA;
- Stuart Levine, Koch Institute for Integrative Cancer Research, Massachusetts Institute of Technology, Cambridge MA;
- Jochen K. Lennerz, Department of Pathology, Massachusetts General Hospital, Harvard Medical School, Boston MA;
- Salil Garg, Koch Institute for Integrative Cancer Research, Massachusetts Institute of Technology, Cambridge MA.
Translated from Classifier les cancers primitifs non diagnostiqués grâce au deep learning