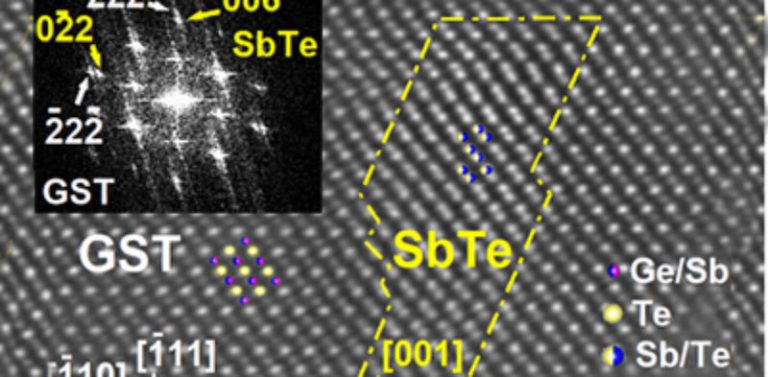
The National Institute of Standards and Technology (NIST) has developed an AI model for materials research. This algorithm, called the Closed-Loop Autonomous System for Materials Exploration (CAMEO ), helps researchers recognize a material as a target. Using this tool, they were able to identify a new component that could be used in photonic devices or for neuromorphic computing.
An algorithm to help materials science
Many scientists are working to discover new materials that can be used in specific applications, such as a lightweight but strong metal for building a car, or an alloy that can withstand high stress and temperature for a jet engine.
But finding such new materials usually requires a large number of coordinated experiments and extensive theoretical research: for example, if a component’s structure changes depending on the temperature of the environment, many experiments will be needed to find out how it behaves. Now imagine that the material does not only depend on temperature, but also on other parameters, the number of tests is multiplied by ten, and the experts cannot perform them all.
In response to this problem, researchers at NIST in the United States have proposed CAMEO, an algorithm to ensure that each experiment conducted can provide a maximum amount of information about the materials to better understand them. The development of this algorithm was described in a paper written by 15 researchers, with Aaron Gilad Kusne as the lead author.
Machine learning to train CAMEO
CAMEO is able to identify a new material by operating in a closed loop: it determines which experiment to perform on a material, performs the experiment and collects the relevant data. It can also ask the researcher for more information before launching the next experiment, such as the crystal structure of the desired material, so that the model can perform the best possible test. At the same time, the expert is informed of all the experiments performed in the loop.
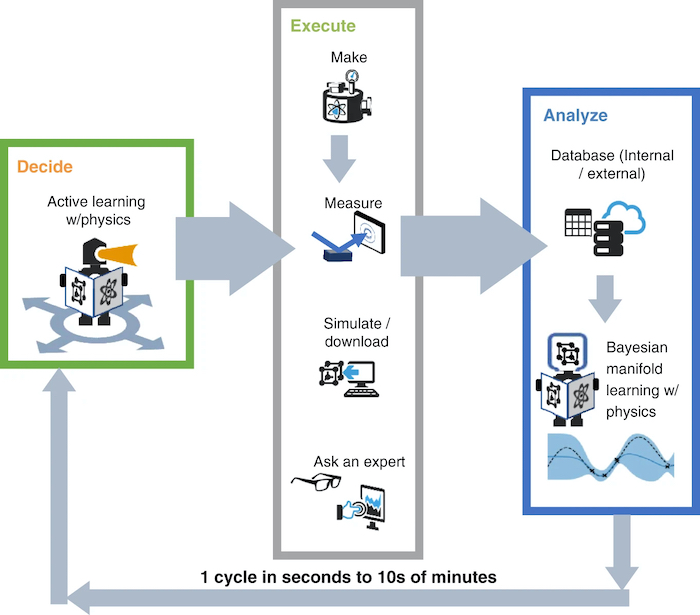
Scientists trained CAMEO using machine learning. To analyze materials, an experiment is performed that requires X-rays. The algorithm is installed on a computer that connects to the X-ray diffraction equipment. CAMEO chooses the material on which the X-rays are focused to study its atomic structure. With each new experiment, the model stores the data and learns it for use in a future test.
The AI is designed to contain all the key principles of materials science, including knowledge of past simulations and experiments. For example, researchers have equipped CAMEO with the basics of phase mapping, which describes how the arrangement of atoms in a material changes with chemical composition and temperature.
The potential benefits of CAMEO
Using CAMEO, the researchers discovered the material Ge4Sb6Te7 (GST467) among the 177 they studied. To achieve this, the model ran 19 experimental cycles that lasted 10 hours. By comparison, it would have taken a laboratory scientist 90 hours to achieve the same result.
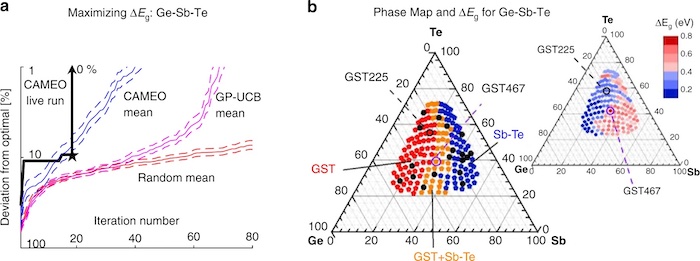
The researchers say that CAMEO could be used for many other materials applications. The code for this algorithm is open source and will be freely available for use by scientists and researchers. The system also allows scientists to work remotely. The team said they are looking to improve the tool and design algorithms capable of solving ever more complex problems.
Translated from CAMEO, un modèle de machine learning capable de reconnaître tout type de matériaux