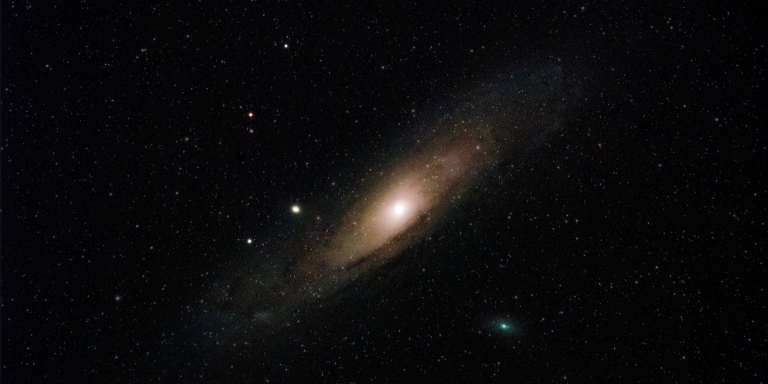
A team from IAS (Institut d’Astrophysique Spatiale) has combined submillimeter observations from the Planck satellite with a method based on artificial neural networks (ANN) to build a tSZ map for the detection of galaxy clusters: MILCANN. The latter allowed them to build a catalog of about 4000 sources that they published and made available to the community.
The Institut d’Astrophysique Spatiale (IAS) is a research laboratory of the CNRS and the University of Paris-Saclay. It gathers 140 people on the site of Orsay (Essonne) and is part of the Observatoire des Sciences de l’Univers de l’Université Paris-Saclay. The scientific and technical teams of the IAS conceive and exploit the data of the instruments of the space missions in astronomy of the CNES, the ESA (European Space Agency) and sometimes NASA. The Planck cosmology mission is one of the major projects of the laboratory, which is the prime contractor of the main instrument.
The Planck satellite
The ESA Planck satellite was launched from Kourou, in French Guiana, on board Ariane 5, at the same time as the infrared satellite Hersschel, in 2009. Since then, it has been mapping the cosmic microwave background radiation, emitted 380,000 years after the Big Bang, when the universe was reduced to a hot, quasi-homogeneous gas. Tiny variations in its temperature provide information on its content, its expansion rate and the properties of the primordial fluctuations that gave rise to galaxies. A first analysis of the data set had been published in 2015, in the form of eight complete maps of the sky that included the polarization of this fossil radiation, that is, the preferential directions in which, at the microscopic level, the light waves vibrate. The detection of galaxy clusters (concentration of at least a hundred galaxies linked together by gravitation) is part of its mission.
The Planck satellite data have already been used three times to produce catalogs of galaxy clusters by detecting the Sunyaev – Zeld’ovich (SZ) effect, which traces the hot gas in galaxy clusters. The technique used was that of multi-frequency matched filtering applied to the six frequencies of Planck’s High Frequency Instrument (HFI).
An artificial neural network to improve mapping
Researchers have already tried to improve the detection of galaxy clusters thanks to the contribution of artificial intelligence: neural networks (Aghanim et al. 2015), then another artificial intelligence technique, based on decision trees, had also been used, allowing to characterize cluster galaxies by estimating their stellar mass and star formation rate (Bonjean et al. 2019).
In this brand new analysis, the authors proceeded in two steps. First, a sky map of the SZ effect was created from the six HFI maps created using Planck. Then, a neural network trained on known clusters was applied to remove pixels from the map with a very low probability of tracing the hot gas. A filter was then used to keep only the sets of pixels tracing galaxy clusters.
Machine learning allowed the researchers to differentiate the characteristic signature of “true” tSZ sources and spurious signals directly from the data using a reference sample of astrophysical sources.
Next, the researchers will employ unsupervised, but physics-guided artificial intelligence algorithms for galaxy clusters to take advantage of their advantage in detecting weak signals and separating the components at the same time.
An R&D study (financed by CNES), as well as a thesis, are in progress on the subject. The final catalog thus obtained contains nearly 4 000 candidates of galaxy clusters, of which only 10% have a probability of being false positives. This new catalog is made available to the community within the SZ galaxy cluster database of IDOC (IAS,http://szcluster-db.ias.u-psud.fr/) and by the CDS (Strasbourg Data Center).
Sources of the article : CNRS.
Translated from Astrophysique : des milliers d’amas de galaxies découverts grâce à l’Intelligence Artificielle