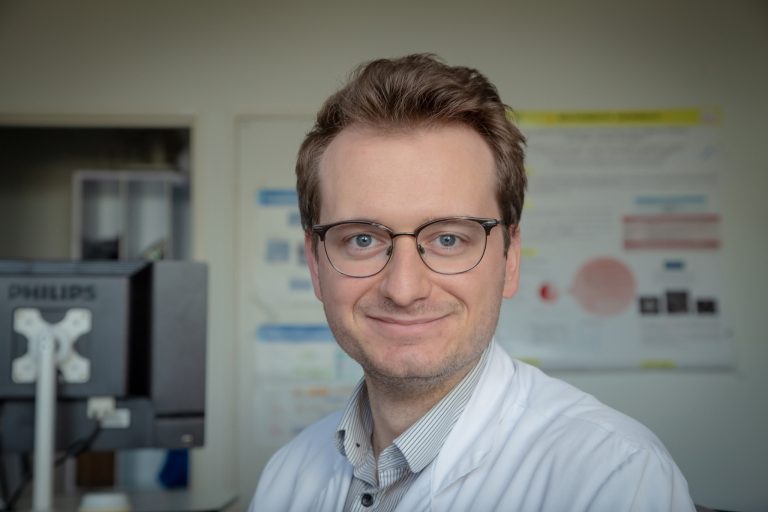
Finalist of the Alsatian edition of “My thesis in 180 seconds”, Stanislas Demuth, a doctoral student in bioinformatics (Inserm – University of Strasbourg) is working on the application of computer technologies to multiple sclerosis (MS) care. We talked with him about his project, augmented intelligence and AI in health, precision medicine tools and the digital transition that the sector is experiencing.
1) Your thesis is about computational approaches for precision medicine in multiple sclerosis, can you tell us about your project?
My PhD aims to develop tools for precision medicine in multiple sclerosis, an autoimmune disease of the brain that affects about 120,000 French people. It is about using digital resources and technologies to assist medical decisions. My reflection is therefore situated between technological development and practice. I regularly alternate between the practice of medicine and scientific research because of a double degree in medicine and science. My field of scientific interest has evolved during this period. Initially focused on immunology, medical practice has made me more aware of applied research and in particular of the challenges of the digital transition of the profession.
“I have always found computerscience to be a tool that allows one to carry out tasks more efficiently or to extend one’s field of competence, whether it be for associative, audiovisual or now medical projects .
With my thesis supervisors, we therefore conceived my doctoral project as a co-supervision and a conciliation of the clinical (professional) viewpoint of Professor Jérôme de Sèze, an expert in multiple sclerosis, and the bioinformatics (technical) viewpoint of Professor Pierre-Antoine Gourraud, an expert in precision medicine. A three-year availability for a science thesis is rare in medicine, where the path is already long, but I am comforted by the feeling that this subject corresponds to me.
2) How does AI fit into your work?
It’s more about augmented intelligence than artificial intelligence. That is, it’s about expanding the physician’s field of expertise rather than completely automating a specific cognitive task. The digitization of health data has exploded the volume of potentially exploitable data, the web allows resources to be shared and the free software movement spreads the technology, notably in the form of Python modules. But moving from massive data to authentic “Big data” requires developing tools to exploit these elements in the action of the business.
For this, the data architecture is more important than the performance of predictive models. The simple ability to access relevant data for a patient and make calculations on the fly during a consultation would already be a small revolution.
Let’s say I’m in a consultation with a patient who has recently declared multiple sclerosis. We have many treatments that slow down the evolution of the disease, in this case, the progression of motor, urinary and cognitive disabilities, etc. These treatments are more or less strong with the progression of the disease. These treatments are more or less strong, with a corresponding risk of adverse effects. Some patients need strong treatments because their disease will be aggressive, others may have a disease that remains dormant for years before reactivating. Which are which?
“That’s the whole challenge of precision medicine: to identify the at-risk profiles to proactively manage the disease and to identify the benign profiles so as not to cause adverse effects unnecessarily.
Currently, we are already looking at tailoring treatments to the patient’s profile. We are building on our previous experience and the results of epidemiological studies that look for risk markers at a population level. Today, when a physician tells a patient that he or she has a 10% chance of being wheelchair-bound in 5 years, he or she is actually extrapolating the average risk in the population or a sub-population of a study. This is an approximation of the patient’s individual risk. It becomes more approximate when there are multiple relevant studies to cross-reference and the physician mentally extrapolates. Because the course of MS is highly variable from patient to patient, this level of precision is not sufficient. However, it is the best granularity possible as long as the experience is only shared at a population level.
The approach of the PRIMUS project, in which I participate, is to share experience at the individual level by reusing data from national scientific registries or therapeutic trials. Neurologists who are experts in the disease have a culture of collecting data from the patients they have been following for years. We are developing software that allows us to access on-the-fly individual data relevant to the patient seen in consultation: in this case, the data of patients similar to mine. Tomorrow’s doctor will be able to explain to the patient that out of 10,000 patients in a reference database, 100 have had his or her profile in the past and that those treated with a particular drug have had the best evolution. And he will be able to do this by showing him the analysis done on the fly on his computer screen. We would therefore be in a situation where the machine reproduces the mental process of the doctor in a more granular, more efficient, more factual, and explained way while serving as a discussion support between the doctor and the patient.
The machine thus underlies a form of trio dialogue between the doctor, the patient and resources: similar patients (known as references), predictive models, knowledge bases, etc. The algorithm identifying relevant reference patients can be as simple as matching. This simplicity promotes physician adoption. There is also a challenge of protecting the identity of the individuals whose data serve as reference patients.
“We hope that an innovative anonymization technique will prevent even indirect re-identification. In short, we are using the machine to delegate the simple and laborious tasks: querying a database, doing a calculation or producing a figure.”
At this stage of the work, the decision-making assistance is a simple description of the evolution data of these reference patients without bringing into play a complex predictive model. In this, we are in an augmented intelligence approach rather than artificial intelligence. Of course, the two are not mutually exclusive! Once this data architecture has been established, we will be able to address more complex tasks such as pattern recognition using machine learning models. It will also be possible to infer prognoses in rare situations where the number of relevant patients is not large enough to be reliable. Matching reasoning may have only limited predictive performance and will need to be refined by predictive models that can take into account more complex, but less explainable, relationships. But we believe that by then, the culture of “numbers in hand” decision making will have progressed.
3) How do you see the post-graduation period? What are your professional goals?
I will do a new rotation, this time from research to medical practice, for the last year of my medical degree. This double curriculum will have lasted 14 years. After that, I aspire to a mixed activity sharing a time of practice and research: a hospital-university career, the theme of which will be to contribute to the digital transition of neurology.
There are so many things to do, that it will undoubtedly go beyond neurology, which could open up great prospects for both doctors and patients.
4) How do you see healthcare evolving in the face of the rise of AI? How do you see the sector in 5-10 years?
I see several hiatuses. A lot of effort is currently put into technological development, less into developing tools for the action of the trade. On the one hand, some players are talking about digital twins, neural networks to predict the evolution of rare cancers, etc. Often, these technologies are based on research data rather than routine data. On the other hand, in everyday life, doctors are still overwhelmed by administrative burdens requiring multiple manual entries of the same data, emergency departments are saturated with polypathological patients whose care is made time-consuming simply because their medical records are not shared efficiently, the ergonomics of hospital and office software still leaves much to be desired, hence the inflation of computer time at the expense of medical time.
This brings me to another opposition between, on the one hand, startups that develop digital tools preferentially for chronic diseases, whose management is subsidiary and relatively standardized. We must recognize the explosion of scientific knowledge that calls for precision medicine and hyperspecialization. On the other hand, the physicians who seem to me to be the most in demand and the most inclined to adopt these tools are those involved in primary care (general medicine and emergencies), whose practice is cognitively demanding, because it is unfilialized by nature. They do not have the possibility of managing this complexity through hyperspecialization since the problems are intertwined in the same patient. This preferential offer for subsidiary care is probably due to a survival bias of startups in these markets.
The emergence of standardization of clinical data and their integration will be the trigger for digital medicine, just as in radiology, where the imaging format has long been standardized: DICOM. Once we have defined the data we are working on, we can start doing machine learning that can impact practice. I see the AI models themselves as a modeling layer on top of this medical “Big data” architecture.
“For me, the real challenge for the profession is to take the lead in digital to design – or at least drive – the development of the tools needed and adapted to the field to manage the contemporary medical day-to-day.”
Although, under current conditions and understandably, many physicians are resistant to the idea of tools that require more screen time, a fortiori during the consultation, I think it is a question of the “toolbox”. Screen time will decrease with efficient tools. The appropriation of digital technology by our profession will open up prospects for data architecture allowing for portability, the automation of time-consuming tasks, the synthesis of medical records, perhaps a renaissance of expert systems, and then the addition of a layer of artificial intelligence: augmented intelligence, in short.
Translated from IA en santé, médecine de précision et sclérose en plaque : entretien avec Stanislas Demuth, doctorant en bio-informatique