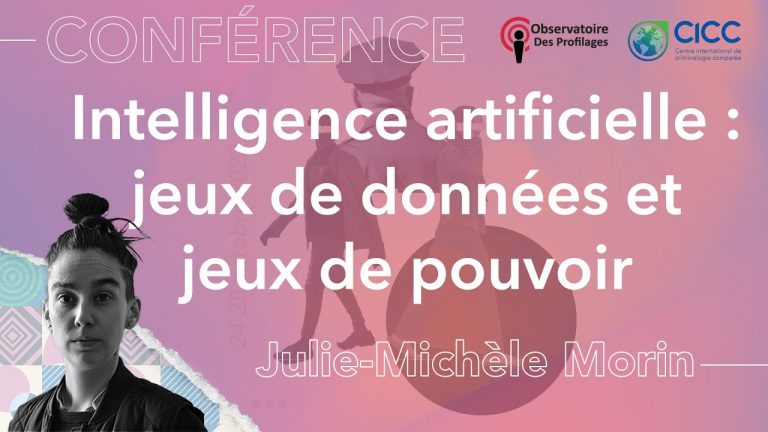
Recently, we devoted an article to the conference ” AI and profiling: ethical and legal risks” by Céline Castets-Renard as part of the ICCC 2022-2023 science week. She emphasized the importance of quality data for training automated systems. Today, we present the second conference of this event: “Artificial intelligence: data games and power games” by Julie-Michèle Morin, also addressing the role of data in the context of profiling.
Julie-Michèle Morin is a doctoral student in French Literature at the University of Montreal. Her thesis focuses on robotics in the performing arts. She mobilizes a technofeminist approach to reflect on the political issues raised by the encounter between the arts, digital cultures and technoscientific devices. She is also a dramaturgical consultant and specializes in media writing.
Data games and their influence
Julie-Michèle Morin first points out that AI systems are always the result of a cooperation between humans (programmers, mathematicians, algorithmicists, computer technicians…) and non-human agents (computer protocols, statistics, mathematical formulas, various automated or semi-automated learning applications…). They are always initiated by humans who can influence the recommendations.
Encoding human values
The speaker addressed the myth of autonomous AIs, capable of exceeding human capabilities, responsible for erroneous results that would not be attributable to humans. This would feed the conception of the so-called technological neutrality: according to the companies or the authorities, they would be more impartial than humans, which allows to trivialize new forms of profiling and discrimination.
The technologization of oppressions
Julie-Michèle Morin refers to facial recognition technology, its inability to authenticate people according to their color in the same way.
Black people are particularly impacted by this technology: by not recognizing them, AI makes people invisible. In the case of identification searches, such as at airports in the United Kingdom, it makes them more visible.
The process steps of AI manufacturing: at what point are biases inserted?
The speaker chose to address the design of an ML algorithm: human biases, stereotypes and prejudices can be encoded when choosing data, labeling it, creating the model to train it.
Insufficient data can be a source of discrimination, too much data on a group, a source of stereotype. Julie-Michèle Morin gives the case of an AI trained on large data sets collected on the web: women were identified as housewives, black men at 10% more than white men as criminals…
Biased data labeling
Data that is manually labeled by a human can be labeled subjectively, it must be framed. When labeled in an automated or semi-automated way, it can carry over historical biases. The example given is that of a recommendation algorithm used by Amazon for recruitment that only retained applications from men because, in the past, the majority of CVs retained were those of men, although this could be motivated by a low number of applications from women.
Biased data analysis
Analysis biases occur when humans create illusory or biased correlations between data sets and the algorithm’s goals. Confirmation biases reflect a pre-existing viewpoint that the AI will reinforce…
Discrimination biases can also be due to the combination of various biases inserted in different steps of the process.
PredPol, a case of predictive justice
PredPol is a software program used since 2012, discontinued this year, to determine where patrols were to occur most frequently. Trained using police records, it recommended that they take place mostly in low-income, majority-black neighborhoods. A study about him showed that he used “dirty datas”.
Like Céline Castets-Renard, Julie-Michèle Morin then considers as an example of predictive justice the COMPAS case, a score for calculating recidivism in relation to certain categories of population, which had the same error rate for both black and Caucasian individuals, but it was not specified that this same rate was in favour of the latter and against the former…
Translated from IA et profilages : la conférence de Julie-Michèle Morin « Intelligence artificielle : jeux de données et jeux de pouvoir »