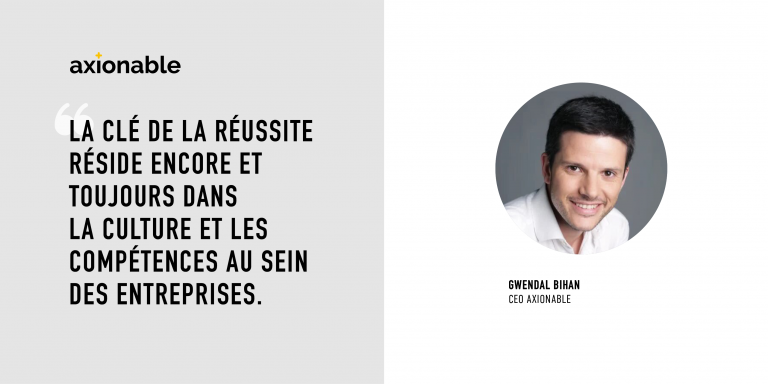
The deployment of AI in our daily lives, both personal and professional, is a source of opportunities and innovation but also presents non-negligible risks in terms of security, privacy, discrimination and environmental impact. To discuss this, we have exchanged with Gwendal Bihan, CEO Axionable, who has evoked with us the importance of trusted AI, by Design, the balance to be found, the regulation but also the situation of the Canadian ecosystem.
The adoption of AI-based solutions is advancing in France, what do you think are the best practices to implement in companies?
Gwendal BIHAN: The key to success still lies in the culture and skills within companies. We advocate on a daily basis for the widest possible understanding of the opportunities for useful innovations as well as the risks linked to AI in companies, and an even greater investment in acculturation and training. Without this understanding at all levels, the adoption of AI remains limited to certain business lines, does not go beyond the Proof of Concept (POC) stage, or may lead to uncontrolled risks. In addition, there are other good practices to keep in mind when it comes to managing AI projects: start with the company’s strategic plan and business issues (and not with data or models), start frugally or adopt a responsible and trustworthy “by design” approach.
While the innovation and opportunities offered by AI are numerous for companies, voices are being raised to warn of the dangers of the technology for freedom, privacy and the environment. How do you position yourself in the face of these risks?
GB: The paradox of AI lies in its infinite potential for useful innovations on the one hand, and on the other hand the generation of potentially unsustainable externalities (bias, carbon footprint, etc.). It is essential for us to manage the balance between these two aspects, with a sustainable purpose for AI on the one hand, and risks and externalities to control on the other.
This balance becomes relative. Let’s take two extreme examples in the field of environment to illustrate this problem: if an AI serves to decarbonize the emissive activities of an industrialist, even with a high training time of the AI model and thus a high CO2 footprint, the net balance will be largely negative at the end, and thus the AI will have a “positive impact” for the planet. On the other hand, if an AI aims to do marketing targeting for carbon products, we can naturally wonder about the net balance of the AI, and the threshold we can collectively tolerate for the footprint of this AI.
International bodies have taken up the topic of AI regulation, and there are multiple opinions between those advocating for more data control and those who would like to see data flow more freely. In this context, how do you think trusted AI can thrive?
GB: The parallel with other societal issues for companies, such as reducing their CO2 footprint, gender parity or QWL (Quality of Life in the Workplace) sheds some interesting light. Studies clearly show that companies that put these societal issues at the heart of their business model, by providing opposable evidence, have a much greater competitive advantage than those who passively wait for regulations to arrive.
We observe that when it comes to trusted AI, this analysis is confirmed. Companies that manage to make it a business issue, get a much higher return on investment than those who will make it a painful compliance project in two or three years when the AI Act comes into force.
Nevertheless, regulation is a good thing to advance these practices throughout the AI ecosystem. A coercive approach, with heavy penalties at the end, is essential for all AI players to transform.
Axionable advocates responsible and trusted AI, how does this manifest itself in your activities?
GB: We have designed a method for developing responsible and trusted AI, which allows us to manage these risks “by design”, from conception to production. Our method is now recognized as a reference on the market and by trusted third parties such as the LNE (IA certification obtained by Axionable in November 2021) or Labelia (advanced label obtained in January 2022).We are already observing it internally, the implementation of these good practices and method gives rise to significant productivity gains.
This responsible and trusted approach is essential to deploy AI in the risky and regulated sectors in which our clients operate. For example, we are working to improve climate resilience at Orano’s nuclear sites, for responsible use of data in financial services with Arkéa, and in the social impact sector for the French Red Cross.
You have also launched Axionable in Canada, what differences / similarities do you see between the two ecosystems?
GB: The Canadian AI ecosystem operates at two speeds. On the one hand, AI research is world class. On the other hand, in the corporate world, the situation is very varied, but in the majority of cases we observe a delay in digital transformation (before even talking about data or AI) in companies, even within some large national groups.
Canada is also much more decentralized than France, like the country itself, with, for example, AI hubs of equivalent level between Montreal, Toronto or Vancouver. They are often in competition with each other and compete to attract foreign investors, especially American and European ones.
As far as regulation is concerned, the market remains much more liberal than in Europe. Paradoxically, this often slows down innovation because in the absence of a clear legal framework, many companies hesitate to use personal or sensitive data for fear of finding themselves in a grey area.
As the equivalent of the RGPD has just been adopted in Europe, the regulation of AI is probably not expected to happen soon on the other side of the Atlantic.
Many thanks to Gwendal Bihan and Marie Geoffroy-Lombard for this interview!
Translated from Adoption de l’IA, bonnes pratiques et IA de confiance by Design : entretien avec Gwendal Bihan, CEO Axionable