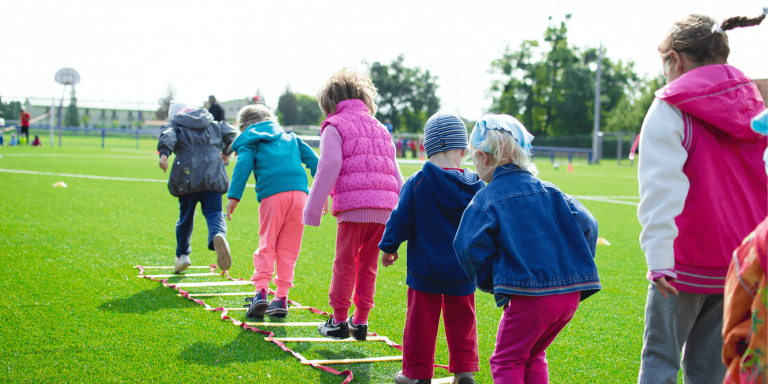
Attention Deficit Disorder with or without hyperactivity or ADHD is a neurodevelopmental disorder, affecting about 6% of school-aged children and 3% of adults. Early treatment is essential, but before that, the diagnosis of ADHD must be made. A team of researchers from the Department of Child and Adolescent Psychiatry and Psychotherapy and the Section of Translational Developmental Neuroscience at the Technical University of Dresden conducted work to identify ADHD, the study titled ” Training a machine learning classifier to identify ADHD based on real-world clinical data from medical records” was published in Nature on July 28.
In children, ADHD manifests itself as a long-lasting attention disorder, which may or may not be associated with impulsivity and/or hyperactivity, and affects an average of three boys for every girl. According to recent studies, 50% of children with ADHD will be cured of this disorder in adulthood, which has been proven by brain scans.
Most of the time, it is the teachers who advise the parents to consult. The treating physician then refers them to specialists: neurologist, child psychiatrist, neuropsychologist, to confirm the diagnosis of ADHD and set up a treatment.
Training an ML classifier to identify ADHD
The diagnosis of ADHD is complex and relies on criteria that are sensitive to subjective bias, which can lead to significant delays in the appropriate initiation of treatment.
Automated analysis based on subjective and objective measures could not only simplify the diagnostic process and reduce diagnostic time, but also improve reproducibility. Recent machine learning studies have been able to distinguish patients with ADHD from healthy controls, however the clinical process requires differentiation between this disorder and other psychiatric conditions.
Researchers at the Dresden Technical Institute trained a linear support vector machine (SVM) classifier to detect participants with ADHD in a population with a wide range of psychiatric disorders using anonymized data from 299 clinical records, achieving 66.1% accuracy.
SVM using single features showed slight differences between features and overlapping standard deviations of the accuracies obtained. Automated feature selection resulted in the best performance using a combination of 19 features.
Data Collection
Collection of a substantial amount of data, although complex, time-consuming, and costly, is necessary to distinguish whether ADHD underlies the particular pattern of symptoms observed as opposed to normal variants of behavior, possible differential diagnoses, or comorbidities occurring in addition to ADHD. Overall, this extensive diagnostic process relies on criteria that are highly susceptible to subjective bias and may result in delays in initiating treatment when effective treatments for ADHD are readily available.
To streamline, shorten, and specify the diagnostic process, it is necessary to identify the most relevant aspects of the data that predict the diagnostic outcome, which is possible through the application of machine learning techniques.
Previous studies based on clinical and/or neuroimaging data have performed automated classifications to distinguish between ADHD and typically developing individuals with classification accuracies ranging from 62 to 89.5%. However, prior to the Dresden team’s study, no study had attempted to apply machine learning to predict whether the diagnosis will be ADHD or “something else” (a standard behavioral variant or other psychiatric diagnosis) across a wide range of clinical conditions.
Because of limitations in cross-sample reliability and validity as well as sensitivity and specificity, neuroimaging or genetic data are not (yet) part of the routine diagnostic process for ADHD. Therefore, readily available behavioral and clinical data, demographic information, subjective symptom ratings, and objective neuropsychological data were used. Indeed, demographic data such as male gender, severe early onset and familial predisposition are associated with a higher risk of ADHD.
Data were from anonymized medical records from the Department of Child and Adolescent Psychiatry and Psychotherapy at the Medical Faculty of the Technical University of Dresden from 2015 to 2020.
Study results
The researchers were able to differentiate children and adolescents with ADHD from those without the disorder with 66.1% accuracy using actual clinical data from hospital records. Excluding demographic characteristics (age and gender) yielded comparable accuracy.
Automated selection using a combination of the 19 most predictive features in the areas of attention and intelligence and symptom assessments performed best, with accuracy potentially further increased using datasets without missing data.
According to the researchers, the highest accuracy achieved in a study of ADHD patients and healthy individuals was 89.5%. Although they used more features than this study, the resulting accuracy is lower, they think this could be due to the fact that many people in their sample had other diagnoses associated with symptoms that may be reminiscent of ADHD (such as attention deficits in depression, increased activity in tic disorders, …).
Multimodal data (especially neuroimaging and genetic data) could improve the recognition of psychiatric disorders through machine learning.
Article Sources:
“Training a machine learning classifier to identify ADHD based on real-world clinical data from medical records”
https://www.nature.com/articles/s41598-022-17126-x
Authors and affiliations
Translated from Une équipe de l’Université Technique de Dresde forme un classificateur ML pour identifier le TDAH