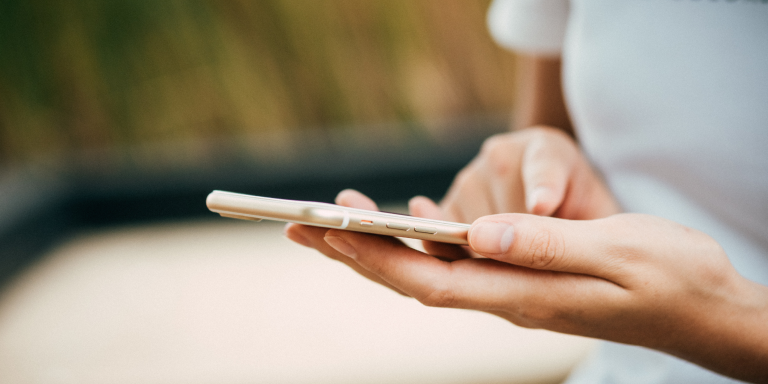
Sometimes we receive advertisements about a product or a service when we have discussed it shortly before with friends or family quietly at home. We can wonder about this strange coincidence: would we be spied on by the voice assistant we own, by some applications on our smartphone, our computer, our connected watch? If this spying is not proven, it is technically possible. A team of three experts in deep learning from Columbia University has developed an algorithm that generates sounds, almost inaudible to humans, that jam the frequencies and thus prevent our own devices from spying on us. They presented their research entitled ” Real-Time Neural Voice Camouflage ” at the last ICLR, (International Conference on Learning Representations, dedicated to deep learning).
Natural language processing (NLP), or automatic language processing (ALP), is a branch of artificial intelligence that allows machines to analyze the human voice to transcribe it into text, understand it, formulate a request or respond to an interlocutor, such as Siri or Alexa. AI algorithms are mainly divided into two groups: recognition and generation. In the case of NLP, recognition consists in analyzing and understanding the sound while generation performs the synthesis. The work of Mia Chiquier, Chengzhi Mao and Carl Vondrick, computer scientists at Columbia University, focuses on these two areas. Their approach is innovative because they have introduced predictive attacks.
The Neural Voice Camouflage Method
The automatic speech recognition models built into almost every smart device have the potential to eavesdrop on conversations. Over the past decade, work has shown that neural network models are vulnerable to small additive disturbances, ambient noise… However, audio streaming is a particularly difficult domain to disrupt because the computation must be done in real time and the software developed so far to counteract eavesdropping was not efficient enough.
The alterations in the sound signal make it almost impossible for a machine to follow the rhythm of a person’s speech. The major issues for the team were optimization and speed: their algorithm, to be efficient, had to be able to predict a change in tone or speed of speech and adapt to it.
Predictive attacks to prevent eavesdropping
The team introduced predictive attacks capable of disrupting any word that automatic speech recognition models are trained to transcribe.
This is actually a signal broadcast by a computer, whose radio frequencies vary according to the speaker’s vocal characteristics, at a frequency of about 16 kHz, which resembles the noise of a silent air conditioner in the background, according to the researchers.
The deep learning algorithm, trained on a large-scale labeled speech dataset, predicts what’s coming next. Then, it will generate a noise model tailored to the prediction, which will make the upcoming speech unintelligible to an automatic speech recognition tool.
Mia Chiquier, assistant professor of computer science and first author of this study, states:
“Our algorithm manages to prevent a malicious microphone from correctly capturing your speech 80 percent of the time. It works even when we don’t know anything about the malicious microphone, such as its location or even the software that uses it.”
The algorithm is only in the prototype stage, the team, which is still working on it, would like to offer it as a downloadable application in various languages.
Article sources:
Real-Time Neural Voice Camouflage
Mia Chiquier, Chengzhi Mao, Carl Vondrick
Columbia University
ICLR 2022 (Oral)
Translated from Une équipe de l’Université de Columbia a développé un algorithme d’IA pour lutter contre les microphones indiscrets